the Creative Commons Attribution 4.0 License.
the Creative Commons Attribution 4.0 License.
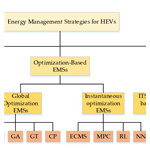
Review article: A comprehensive review of energy management strategies for hybrid electric vehicles
Yuzheng Zhu
Xueyuan Li
Qi Liu
Songhao Li
Yao Xu
In order to prevent the aggravation of global environmental problems, all industries are facing the challenge of green development. In the automotive field, the development of “new-energy vehicles” (plug-in electric vehicles) is particularly necessary. Hybrid electric vehicles (HEVs) have been proven to be an efficient way of solving environmental and energy problems. As the core of HEVs, the energy management strategy (EMS) plays an important role in fuel economy, power performance, and drivability. However, considering the randomness of actual driving conditions, there are great challenges involved in the establishment of an EMS. Therefore, it is critical to develop an efficient and adaptable EMS. This paper presents a systematic review of EMSs for HEVs. First, different issues that can affect the performance of EMSs are summarized. Second, recent studies on EMSs for HEVs are reviewed. Third, the advantages and disadvantages of different categories of EMSs are compared in detail. Finally, promising EMS research topics for future study are put forward.
- Article
(4588 KB) - Full-text XML
- BibTeX
- EndNote
In the world today, the greenhouse effect is becoming more and more serious, and the global energy crisis is intensifying. In addition, the quantity of vehicles is increasing year by year. Because of the severe situation, governments around the world have published policies for the development of “new-energy vehicles” (plug-in electric vehicles) to promote the transformation and development of the traditional automobile industry. Under the current industrial conditions, hybrid electric vehicles (HEVs) have become one of the most important divisions of new-energy vehicles with respect to solving energy and environmental problems. Compared with electric vehicles (EVs), HEVs can get rid of the limitations of battery technology, improve endurance with lower energy consumption and emissions, realize the complementary advantages of multiple power sources, and improve the integral performance of the vehicle.
A HEV is a nonlinear, multi-input, multivariable complex system with two or more power sources. One power source is a traditional internal combustion engine (ICE), and the other power source is a fuel cell, generator, electric motor (EM), or other components (Miller, 2010). One or more power sources provide the demand power for HEVs according to different working conditions. A HEV includes the mutual conversion process of electrical energy, mechanical energy, and internal energy (Xue et al., 2020). In general, HEVs can be divided into series, parallel, and power-split types (Sabri et al., 2016). In series-type HEVs, the ICE does not directly drive the vehicle but instead provides energy to the EM and the battery through the ICE generator. This structure has fewer driving modes, and there is no dynamic coupling problem between various power sources, so the control process is relatively simple (Miller, 2006). For the parallel-type and power-split-type HEVs, multiple power sources can be driven individually or jointly according to the working conditions (Singh et al., 2019). A coupling mechanism between the power sources is applied to realize the power output under different working conditions, so the control process is more complicated (Husain 2005). The coupling mechanism can be divided into a torque-coupling type, a speed-coupling type, and a power-coupling type (Krithika and Subramani, 2018). It can be adopted to adjust and optimize the working state of each power source, thereby improving the performance of the vehicle (Xiang et al., 2010).
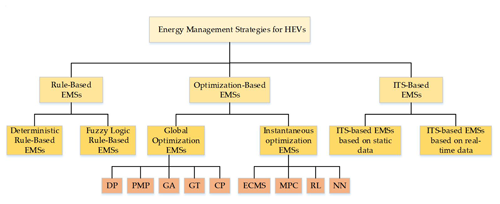
Figure 1Types of energy management strategies (EMSs) for hybrid electric vehicles (HEVs). Please see Appendix A for a full list of the abbreviations used in the figures.
As the core technology of HEVs, the energy management strategy (EMS) directly affects the economy, power performance, driving performance, and reliability of the vehicle. The EMS solves the problem of energy distribution between different power sources (Wang et al., 2017a; Hannan et al., 2014). According to the state of charge (SOC), the driver's pedal signal, the characteristics of the ICE and the EM, and the power demand of the vehicle, the power or torque distribution of each power source is solved to improve the fuel economy of the vehicle (Sulaiman et al., 2015; Zhao and Guo, 2016).
In recent years, as the level of research has increased, various EMSs have been developed and have gradually matured (Panday and Bansal, 2014). At first, scholars developed rule-based EMSs based on experience. Following this, global optimization EMSs, based on dynamic programming (DP) and Pontryagin's minimum principle (PMP), and instantaneous optimization EMSs, based on the equivalent consumption minimization strategy (ECMS) and model predictive control (MPC), were successively proposed. With the emergence of various intelligent algorithms and the continuous progress of EMSs, genetic algorithm (GA), game theory (GT), convex optimization (CO), reinforcement learning (RL), and neural networks (NNs) have been gradually applied in EMSs. In addition, with the continuous development of the intelligent transportation system (ITS) and “Internet of Vehicles” (IoV) technology, the information interaction between vehicles, roads, and people has gradually deepened. Information based on the ITS has also been gradually applied to EMSs, as it extends the energy management issue from a vehicle to the transportation system. With the development of HEV technology, the existing EMSs have been updated and optimized, and EMSs based on multi-method fusion are continuously proposed. The performance of EMSs is continuously being improved, and multiple efficient EMSs are gradually being applied to HEVs. The specific types of EMSs for HEVs are shown in Fig. 1 (F. Zhang et al., 2020). Many scholars have conducted extensive and in-depth research on HEV energy management, and many representative review articles have been published that comprehensively summarize EMSs and can be used as guidance.
The characteristics of the different reviews are summarized in Table 1.
With the development and application of the ITS, EMSs of HEVs are gradually separated from the limitation of one vehicle and are oriented toward optimization based on the whole traffic system. Therefore, on the basis of traditional classification methods, EMSs are divided into rule-based, optimization-based, and ITS-based strategies. This paper is organized as follows: in Sect. 2, limitations of HEV driving conditions and the issues considered in EMSs are summarized; in Sects. 3–5, the research status of rule-based EMSs, optimization-based EMSs, and ITS-based EMSs is reviewed in detail; finally, in Sect. 6, promising EMS research topics for future study are briefly put forward.
Energy management is a key issue in the research of HEVs and is fundamental for the efficient and clean operation of the vehicles. Energy management aims to solve the problem of energy distribution among different HEV power sources. Based on different HEV driving conditions, the output of each power source is reasonably distributed to meet the driving demands of HEVs; the performance demands of the vehicle economy, emissions, and other aspects; and to extend the service life in the meantime (Martínez et al., 2017; Yu et al., 2006). During actual driving, HEVs are divided into different working conditions according to the working state of the ICE and the EM. Under different working conditions, the power demand of each component is quite different (F. Zhang et al., 2019); therefore, the role of the EMS is to fully exploit the respective advantages of the ICE and the EM so that most of the working parts can operate in the high-efficiency range in order to improve the efficiency of the vehicle.
The energy management issues not only take the working range and emissions of the ICE into account but also the efficiency of the EM, the battery, and the transmission system. In the process of energy management, modeling and optimization should be carried out according to one or more optimization objectives (Hannan et al., 2014). Irrespective of the kind of EMS adopted, in addition to meeting the vehicle driving demands, it needs to meet the safety performance demands. Therefore, the implementation of the EMS needs to meet the following boundary conditions:
where Te denotes the torque of the ICE, ωe denotes the speed of the ICE, Tm denotes the torque of the EM, and ωm denotes the speed of the EM.
In the research regarding EMSs, the energy management issues can be transformed into a cost function problem with different optimization goals (Gu et al., 2019). Generally, the energy management issues can be transformed into the energy management issue of the SOC, the energy management issue of equivalent fuel consumption, the energy management issue of the instantaneous condition, and the energy management issue of emissions.
2.1 The energy management issue of the SOC
The battery is the key component of HEVs, and the SOC is closely related to the battery capacity and charge–discharge characteristics. If the SOC is too high or too low, it will affect the performance of the battery. Thus, it is necessary to control the working range of the SOC, and many EMSs take the SOC as one of the optimization goals, aiming to optimize the working range and prolong the service life of the battery. Therefore, the SOC can be regarded as a threshold value that limits the operation interval of the EMS; it can also be regarded as a weighting item for calculating the cost function of the HEV. In addition, frequent charging and discharging will affect the life of the battery, thereby affecting its output power. X. Hu et al. (2020) state that the loss and degradation of the battery can affect the accuracy of the EMS; thus; the state of health is introduced into the EMS. The expression in the cost function is as follows:
where Cbat,j denotes the cost item of the battery degradation in the cost function, which constitutes the cost function of the EMS and other items; soh(tk) denotes the state of health of the battery; Mbat denotes the cost of the battery system; Q denotes the nominal capacity of the battery; N denotes the number of cycles at the end of battery life; Atol denotes the total discharged Ah (ampere hour) throughput; and i(tk) denotes the current of the battery.
2.2 The energy management issue of equivalent fuel consumption
While calculating the cost of a HEV, the energy consumption is usually converted into the sum of the fuel consumption of the ICE and the power consumption of the EM, which can be regarded as the equivalent fuel consumption. Generally, the cost function is applied to describe the equivalent fuel consumption. The proportion of the fuel consumption of the ICE and the power consumption of the EM in the cost function can be adjusted according to the actual driving conditions to make the control more accurate. This energy management issue can be described as follows (Wang et al., 2017a):
where J denotes the energy consumption cost function of the HEV in the period from tk to tk+p, including the fuel consumption cost f(t) and the power consumption cost g(t); α1(t) denotes the weighting coefficient of the fuel consumption; and α2(t) denotes the weighting coefficient of the power consumption. The equivalent factor (EF) can be defined as EF(t) and can convert the power consumption cost into the fuel consumption cost. The EF can be regarded as a fixed value designed based on experience, or it can be designed as an adaptive EF that is adjusted in real time according to the characteristics of the EM and the battery. The optimization goal of this energy management issue is to minimize the cost function over a period or instantaneously.
2.3 The energy management issue of instantaneous conditions
During the HEV driving process, the vehicle may experience instantaneous driving conditions, such as start–stop and gear shifting, over a long period. Frequent starting and stopping of the ICE will cause an increase in fuel consumption. Therefore, the fuel consumption of instantaneous conditions is usually converted into the cost function. According to the model of instantaneous conditions, the fuel consumption of shifting, frequent starting and stopping of the ICE, and starting and braking conditions are considered. They can be regarded as the weighted terms in the cost function, which improve the accuracy of the cost function and can better reflect the fuel consumption during actual driving. In Yan et al. (2012), the fuel consumption during the ICE start–stop process is added into the EMS, which forms the cost function along with the SOC and equivalent fuel consumption.
Here, the first term denotes the equivalent fuel consumption of the HEV within time interval tp, the second term denotes the equivalent fuel consumption of the SOC within the time interval, the third term denotes the equivalent fuel consumption of the ICE start–stop process, g(t) can be defined as the function of the SOC, key_on(t) denotes the start or stop state of the ICE, and α3(t) denotes the weighting coefficients of the fuel consumption cost caused by the start–stop state of ICE in the cost function.
In Y. Qi et al. (2017), experimental data from the ICE are applied to solve the dynamic response model of the ICE and the controller, and they are introduced into the cost function along with the output characteristics of the EM in order to reduce the impact of ICE instantaneous characteristics on energy management issues.
As the perdition control model, tk denotes the time at the kth prediction horizon, and tp is the time duration of the prediction horizon; ωωe(t), ωte(t), ωωA(t), and ωDe(t) denote the respective weight function of the reference ICE target speed, reference target torque of the ICE , reference target speed of EM, and reference fuel consumption rate of the ICE (which can also be regarded as the penalty functions); , , and denote the reference values of the respective ICE speed, output torque of the ICE, and EM speed; De(t) denotes the reference index of the fuel consumption rate of the ICE.
2.4 The energy management issue of emissions
The emergence of HEVs has stemmed from the requirement to save energy and reduce emissions. The EMS is not only related to the dynamic performance but also directly affects the emission performance of vehicles. Therefore, emission indicators and emission control are integrated into the study of energy management issues. The emission of pollutants such as CO2 and NOx is usually introduced into the cost function through certain methods, and the cost function is constructed to explore the multi-objective optimization of energy consumption and emissions. (Nüesch et al., 2014a).
where ERn denotes the calculated value of specific emissions (NOx), and μ is the weighting coefficient of the emission performance. A larger value of μ indicates that the cost function pays more attention to emission performance, whereas a lower value of μ indicates that energy consumption is more important in the search for the instantaneous optimal solution.
The rule-based EMS is one of the most important EMSs applied to HEVs. The principle of this EMS is relatively simple, and it does not require the use of a complex algorithm; therefore, it has been widely used in early-stage engineering (Jalil et al., 1997). At present, there are two main forms of rule-based EMS: one is the deterministic rule-based EMS, which regulates the working state of various vehicle parts according to different driving demands and the working range limits; the other is the fuzzy logic rule-based EMS, In the latter EMS, according to multi-input and time-varying characteristics, the advantages of fuzzy logic control are integrated into the EMS, the membership function (MF) of state variables and the rate of change of state variables are established, and the fuzzy logic rules are determined for energy management and the SOC regulation. The rule-based EMS is based on experience, the driving mode, and a static map, and it is widely used and less affected by the external interference. The idea is simple and easy to implement, and it can be designed based on the existing vehicle control concept. In addition, modern algorithms such as NNs and GA can be used to optimize the EMS, which can improve its adaptability, to a certain extent, with respect to dealing with complex dynamic changes. However, the engineering experience directly determines the performance of the EMS, and it is difficult to obtain the optimal control effect.
3.1 The deterministic rule-based energy management strategy
In this EMS, the deterministic rule was established based on the parameter characteristics of each component, existing engineering experience, and research results. It can adjust the working status and power distribution according to the driving demands and the working conditions of each component. The main idea of this EMS is to use the EM to adjust the working range of the ICE so that the ICE is always working in the high-efficiency range. In addition, it is necessary to combine the battery to select a suitable driving mode for the HEV. The input variables of this EMS are mostly the demand power and the SOC. It has an optimization effect for any driving condition, with certain adaptability. Generally, the deterministic rule-based EMS can be divided into the logic threshold strategy and the “following” strategy. The logic threshold strategy takes the limiting conditions, such as the ICE working point and the SOC as threshold, and adjusts the working state of each component according to the threshold. The following strategy tracks one or more operating parameters and uses them as a basis to adjust the operating status of each component. The tracking parameter is mostly the output power of the ICE. Although, there are also EMSs that set the speed or the load as the tracking parameter.
3.1.1 The logic threshold strategy
The working modes can be divided into the EV mode, the charge-depleting (CD) mode, and the charge-sustaining (CS) mode, according to the SOC threshold: in EV mode, the EM is driven separately; in CD mode, the working state of the ICE and EM is adjusted according to the high-efficiency interval of the ICE; and in CS mode, it is necessary to maintain the SOC around a threshold. In addition, one can switch between the CD and CS modes; thus, the working point of the ICE is always in the efficient range, and the working state of the EM is judged according to the driving demands (Peng et al., 2015). The mode-switching control of the logic threshold strategy is shown in Fig. 2.
In Y. Liu et al. (2019), a logic threshold strategy based on the efficiency range of the ICE and the optimal operating range of the battery was proposed to keep the SOC at a high level and improve the efficiency of the ICE. In Asghar et al. (2018), an EMS based on the Atkinson cycle ICE was established, and the driving mode was determined according to the speed and torque demand. Based on the fuel cell hybrid power system, the SOC of fuel cells and lithium batteries and the voltage state of supercapacitors were set in Y. Wang et al. (2019), and the logic threshold strategy was proposed to assign the different working states of various components. The system can utilize the charge–discharge limitations of power capacity and residual energy to extend the service life. In Hao et al. (2016), the working range of the ICE and battery, the minimum throttle opening of the ISG (integrated starter generator) motor and EM auxiliary conditions, and the minimum vehicle speed of the EM driving conditions were used as the threshold. The direct algorithm can transform the EMS into a direct optimization problem of seven-dimensional parameters. In Jeoung et al. (2019), the start–stop of the ICE was controlled according to the threshold of demand power, torque, and speed, and the battery charge–discharge process was determined according to the SOC threshold in which the charge–discharge speed can be used as a threshold. In Xia and Zhang (2015), an EMS based on the quadratic performance index which was independent of future driving conditions was proposed. The operating conditions of the ICE and EM were adjusted according to the speed and SOC as well as the expected speed and SOC values. In Padmarajan et al. (2016), an EMS based on mixed rules was proposed. The driving information and estimated vehicle trip energy were combined with a blended charge-depletion strategy to reduce the ICE start–stop times. In Zhou et al. (2018), the working state of the ICE was determined according to the SOC threshold, and DP was applied to determine the optimal trajectory of the ICE and the corresponding SOC threshold.
3.1.2 The following strategy
The following strategy can be divided into the power-following strategy (PFS), the speed-following strategy (SFS), and the load-following strategy (LFS). The essence of the PFS is to ensure that the output power of the ICE and EM as well as the vehicle load power match the sum and maintain ICE function within the highly efficient range (Li, 2019). The SFS adjusts the driving conditions in real time according to the speed. The LFS mainly adjusts the charge–discharge process of the battery according to the power demand and indirectly adjusts the working state of each component. At present, the most widely used strategy is the PFS.
In Li (2019), discrete speed switching and the best fuel consumption curve of the PFS were compared. The performance of the best fuel consumption curve of the PFS was better. In Zuo et al. (2009), a full-vehicle mode transition algorithm was proposed to switch vehicle modes, and a PFS based on the minimum fuel consumption curve of the ICE was adopted using the planetary row kinematics limit model. In Luo et al. (2019), the PFS was combined with two HEV DC-line voltage control strategies (CVPI, complete zero voltage switching control, and PZVS, persistent zero voltage switching control). According to the minimum mass point of the equivalent fuel consumption, the comparative study showed that the PFS PZVS had better fuel economy. In B. Zhang et al. (2020), an adaptive smoothing PFS based on the optimal efficiency graph was proposed, dividing the demand power into the trend and the fluctuation parts. The trend part was provided by the ICE, and the fluctuation part was provided by the supercapacitor. In Chen et al. (2019), according to the closed solution of optimal power diversion, the truncated battery-following strategy was developed to reproduce the global optimization solution of DP. In Geng et al. (2019), an on/off PFS optimized by fuzzy logic was proposed for a fuel cell HEV and was used for extended controllers. In Mohamed et al. (2019), two following strategies were proposed to select the driving mode of the HEV: one was the SFS, which selected the start–stop of the ICE according to the vehicle speed, and the other was the LFS, which selected the operating mode according to a set power threshold and the SOC. This comparative research found that the energy-saving effect of the LFS was better. In Bizon (2019), an EMS based on the LFS and real-time optimization was proposed to evaluate the fuel cell economy and efficiency performance indicators. Weighting coefficients were applied to mix performance indicators into an optimization function.
Table 2Summary of exemplary works on deterministic rule-based EMSs
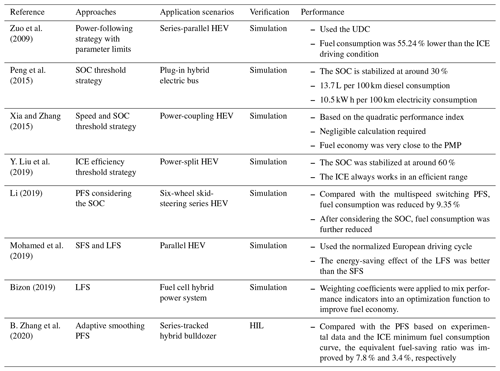
HIL denotes hardware in loop. UDC refers to the Urban Driving Cycle.
The characteristics of different deterministic rule-based EMSs are illustrated in Table 2. The deterministic rule-based EMS has a simple control process, convenient parameter adjustment, good robustness, and good stability. However, this EMS does not provide the best performance; instead, it provides a range of preliminary optimization in a specific driving cycle or instantaneously. In addition, the control rules of the deterministic rule-based EMS are mainly based on engineering experience and test data, which have many uncertainties and cannot meet the time-varying requirements of HEVs. Moreover, there are obvious limitations in the actual control process, and this method cannot entirely utilize the energy-saving advantages of HEVs. Therefore, in the study of deterministic rule-based EMS, optimizing multiple control parameters is of great significance to improve vehicle performance.
3.2 The fuzzy logic rule-based energy management strategy
Fuzzy control is a kind of control method with strong robustness, easy adjustment, and strong adaptability that can imitate the uncertain thinking mode and logic of the human brain. Fuzzy control uses the MF to reason some systems with strong uncertainty, nonlinearity, or an unknown mathematical model; to solve problems that are difficult to solve using conventional methods; and to simplify the calculation process. The main process of fuzzy control is “fuzzification” and “defuzzification”, and the core component is the fuzzy logic controller. The input signals are transmitted to the fuzzy logic controller where they are fuzzified, and the fuzzy results are then obtained according to the MF. Following this process, the fuzzy results are defuzzified to obtain the output signals used for precise control (Jager, 1995). Generally, the input signals of HEVs are the power demands obtained from the pedal signal and ground information, combined with the status parameters, the working state, and the output power of each component, which are obtained through fuzzification and defuzzification (Li, 2008). The basic principle of a fuzzy logic rule-based EMS is shown in Fig. 3.
The following outlines the application of traditional fuzzy logic rule-based EMSs to HEVs. In Hemi et al. (2014), an EMS based on fuzzy logic was proposed that took the demand power and SOC as the input for the fuzzy logic controller and considered the influence of regenerative braking on the battery in the MF design process in order to meet the power demand and protect the battery. In Ma et al. (2019), an EMS based on fuzzy logic that took the SOC and demand torque as inputs was proposed to optimize the torque output. The MF was established according to the SOC, and the output torque of the ICE and the auxiliary output of the EM were obtained. According to the real-time transmission efficiency of HEVs, a fuzzy logic rule-based EMS was proposed based on the optimal working line of the ICE and mechanical point control strategy in S. Wang et al. (2019a) to realize the synchronous improvement of transmission efficiency and fuel economy. In Mahyiddin et al. (2016), a triangular MF was established for the battery charge–discharge process and power split. Fuzzy logic was used to distribute the output power between various power sources to compensate for the power flow performance. In Denis et al. (2015), an EMS based on fuzzy logic was established according to past and current driving information as well as the expected travel distance, and DP was adopted to optimize it. In addition, the driving information was used to achieve adaptive control of different driving conditions. In Singh et al. (2020), the regenerative braking process was introduced into the fuzzy logic controller, requiring the ICE and EM to work in an efficient interval. The MF was designed based on the driving demands and fuel economy.
With deepening research into HEVs, the adaptability of EMSs has become more and more critical, and the adaptive fuzzy logic rule-based EMS has been proposed and gradually applied. In X. Zhang et al. (2017), a fuzzy EMS based on the optimization algorithm of the adaptive neural fuzzy system was proposed that took the demand torque of the clutch and SOC as input and the torque of the ICE as output. Gradient search technology was applied to adjust the weight of each layer and the output results so that the least squares method between the actual output and the expected output could reach the minimum. In Tian et al. (2018), an adaptive fuzzy logic rule-based EMS for hybrid city buses was established according to the optimal SOC curve. An NN was used to learn the best SOC curve and realize the planning and control of the battery working state according to future driving information from the online ITS and navigation system. In Shen et al. (2020), the efficiency and power change rate of the fuel cell were considered to balance the load of the power system, and the power slope of the fuel cell was limited to prevent the abrupt change. An incremental fuzzy logic EMS was proposed to ensure that the fuel cell was working in the high-efficiency range and to prolong its life. In Sabri et al. (2018), a dedicated fuzzy logic EMS for “through-the-road” HEVs was proposed that determined the power flow based on the global discharge rate obtained from the current vehicle speed, SOC, and remaining travel distance and also gave priority to the output from the electric drive system. In Sölek et al. (2019), an EMS combined with online and off-line algorithms was proposed. The online algorithm used fuzzy logic to select the control method and the driving mode, and the off-line part was established based on the average consumption data in EV mode.
With the advancement of computer science, various real-time algorithms have been proposed, and the fuzzy logic EMS based on algorithm optimization has gradually been applied. In Shi et al. (2018), the energy management issue was described as a predictive control problem. A Markov chain was used to solve the power demands and speed in the predictive layer. A fuzzy logic controller was used to achieve optimal tracking of the ICE speed in order to ensure that the ICE could realize the desired power stably. In Peng and Xie (2017), the MF of the EMS based on fuzzy logic was optimized using the GA to solve the SOC maintenance and power distribution problems. In addition, the CO and NOx emissions were taken into consideration. In Meng et al. (2017), a fuzzy logic EMS based on GA optimization was proposed. The GA was used to optimize the MF based on historical data, which effectively prevented the EM from generating peak torque, and the ICE mostly worked in the efficient zone. In Singh et al. (2021), an EMS based on fuzzy logic and Ehrman NNs was proposed. The optimized fuzzy logic controller's input was the demand torque, the SOC, and regenerative braking, which aimed to maximize fuel economy while maintaining battery health. In Liu et al. (2017), an EMS based on fuzzy logic and RL was proposed. Fuzzy logic and Q-learning were used to realize speed prediction, and RL was used to learn the transition probability of power demand. In Q. Xu et al. (2018a), a dual-optimization fuzzy logic EMS based on the GA and DP was proposed: the GA was used to optimize the MF, and DP was used to optimize the fuzzy logic controller. The braking energy recovery was considered. In Mohammad et al. (2020), a fuzzy logic EMS based on the social spider algorithm was proposed to adjust the scaling factor in the MF in real time to reduce speed-tracking errors.
Table 3Summary of exemplary works on fuzzy logic rule-based EMSs.
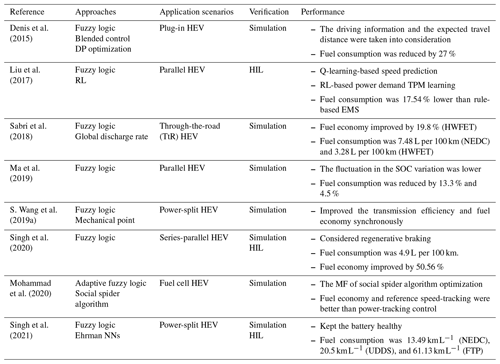
TPM represents transfer probability matrix. HWFET refers to the Highway Fuel Economy Test cycle. NEDC refers to the New European Driving Cycle. UDDS refers to the Urban Dynamometer Driving Schedule. FTP represents Federal Test Procedure.
The characteristics of different fuzzy logic rule-based EMSs are illustrated in Table 3. In the control process, the fuzzy control is not completely dependent on the precise mathematical model, which can greatly reduce the amount of calculation required. It also has high efficiency, good robustness, and good economy. However, when designing fuzzy rules and the MF, there are no certain rules to be followed. Instead, it is an experience-based EMS that cannot take full advantage of the energy-saving and emission reduction potential of HEVs; therefore, it is not the optimal solution in the scientific sense. In addition, the fixed control law leads to poor dynamic characteristics of the system, which makes it difficult to realize real-time control and optimization. Therefore, in the current research process, the GA, NNs, and RL are often used to optimize fuzzy logic in order to improve the real-time application and controllability of fuzzy control.
In the optimization-based EMS, the cost function is designed to combine the structural parameters of each vehicle component and constraint. This EMS minimizes the cost function to optimize the control objective. The control objective is fuel consumption. Some scholars also integrate parameters such as the battery power consumption, emissions, and the battery health level into the cost function for multi-objective optimization. In general, the optimization-based EMS can be divided into two categories: one is the global optimization EMS, which takes the operating cost of the whole driving condition as the optimization objective and utilizes the optimal control theory based on historical data to conduct global optimization, and the other is the instantaneous optimization EMS, which takes the instantaneous fuel consumption and other parameters as the optimization objectives. Combined with the instantaneous parameters, it controls the working state of each power source and instantaneously minimizes the cost function under unknown driving conditions.
4.1 The global optimization energy management strategy
In the research regarding the global optimization EMS, representative methods include the DP-based EMS and the PMP-based EMS. In addition, intelligent algorithms such as the GA, GT, and CO are also applied to the global optimization EMS. A comparison of these different methods is shown in Table 4, which illustrates the pros and cons of each method. The global optimization EMS is usually optimized for a fixed cycle of driving conditions, which has a certain theoretical guiding significance and is suitable for HEVs with relatively fixed working conditions. However, under unknown driving conditions, the global optimization solution is not the optimal result in practical sense. Therefore, it is suitable to be used as a reference for the control effect of other EMSs.
4.1.1 The DP-based energy management strategy
DP is a mathematical method to solve the optimization of the multistage decision process and was applied to the HEV energy management issue around 2000. The entire interval of the energy management issue is discretized into multiple intersections by DP. Appropriate control variables are then selected according to each intersection. The solution of the first intersection is used as a reference for the solution of the next intersection, and the solutions that could reach the optimal value are retained until the last intersection. DP is often used to optimize conventional fixed driving routes, such as hybrid electric buses. In DP-based EMSs, the input parameters are the driving cycle information and the constraints. The energy management issue is discretized and can be converted into the problem that calculates all paths from beginning to end. The global optimization result is the sum of the results of each step. The principle of a DP-based EMS is shown in Fig. 4.
However, it is impossible to predict the whole driving process under actual driving conditions, and the calculation is heavy and time-consuming, so it cannot be applied to real-time control (Bertsekas, 1995). Therefore, the adaptive DP and the heuristic DP have been proposed. Combined with historical information, they can improve the real-time performance of the traditional DP method. In recent years, the stochastic DP (SDP) was proposed based on DP, which discretized the driving conditions through power demand and speed, and established probability transfer matrices of the current and next moment based on a Markov chain in order to estimate the power demand and other parameters at the next moment (Birge and Louveaux, 1997). This method is based on historical driving data and does not require a complete driving cycle. It can obtain the optimal control rate and realize real-time control to a certain extent. However, there is still a certain deviation between the predicted demand and the actual demand by the Markov chain. The adaptability of SDP to multiple working conditions still needs to be improved.
Due to its excellent performance with respect to solving the multistage decision optimization problem, DP has been introduced to EMSs. In H. Li et al. (2019), a DP-based EMS was proposed that considered the SOC as the state variable, the transmission ratio of continuously variable transmission (CVT), and the electric torque distribution between power sources as the output to solve the minimum value of total fuel consumption. Due to the large computation burden and long computation time of the DP-based EMS, a respective local linear approximation and a quadratic spline approximation were used in Larsson et al. (2015) to shorten the calculation time and reduce the storage pressure. In Delkhosh et al. (2020), DP was adopted to find the best operating mode at each point in the HEV operating region. The EMS is established according to the optimal operating region to realize the conversion of the operating modes. In Pam et al. (2019), the influence of ramp resistance on fuel economy was accurately quantified using DP. An EMS considering ramp resistance was proposed to reduce the fuel consumption calculation error caused by ignoring the ramp. With the aim of applying a DP-based EMS to plug-in HEVs, Wang et al. (2015) overcame the numerical problems between the optimization accuracy and calculation burden and further exploited the energy-saving potential of DP-based EMSs.
However, DP-based EMSs have some limitations under unknown driving conditions. Therefore, scholars have proposed adaptive DP and heuristic DP based on traditional DP. In Kalia and Fabien (2020), an EMS based on distance-constrained adaptive real-time DP for extended-range electric vehicles was proposed. This strategy monitored the SOC deviation from the calculated optimal state. It recalculated the optimal parameters accordingly to approach the real-time control and improve the adaptability and fault tolerance. In Zheng and Mi (2009), an adaptive DP-based EMS that combined DP with fuzzy logic was proposed. Firstly, the optimization results were obtained by DP, and the efficiency of each power point of the ICE was analyzed to reduce the degrees of freedom of the EMS. The MF was then established according to the ratio of the ICE power to system power, speed, acceleration, and SOC. In Liu et al. (2019), a heuristic DP-based EMS was proposed for the online optimization of HEVs. A back-propagation NN (BPNN) was adopted to build a vehicle model that reflected the actual dynamic process of HEVs. According to the dynamic model, an online algorithm was used to optimize the energy management process of heuristic DP. In Li and Görges (2019a), a heuristic DP-based EMS was proposed that was combined with the adaptive cruise control in order to ensure the distance from the vehicle in front. Moreover, an action-dependent heuristic DP was used to realize active distance control. This EMS can adjust internal vehicle parameters online to deal with system disturbances and achieve the economy and drivability requirements. In Li and Görges (2019b), the NN-based shift control was combined with the action-dependent heuristic DP-based power distribution control, and a real-time adaptive EMS was proposed that supported the online learning of the controller and could significantly reduce the calculation load of DP and improve the calculation speed.
In addition, SDP has also been applied to EMS to improve real-time performance and adaptability. In Opila et al. (2012, 2013), an EMS based on the shortest-path SDP was proposed in which the driving cycle was modeled as a finite-state Markov chain. The cost function consisted of a weighted sum of fuel consumption and drivability losses from shift and ICE-switching events. By changing the weight of each component, both drivability and fuel economy could be improved. In Qin et al. (2017), an SDP-based EMS for a pre-transmission single-shaft torque-coupling parallel HEV was proposed. The driver's demand torque was modeled as a Markov process to represent the uncertainty of future driving conditions. In Elbert et al. (2015), an SDP-based EMS was proposed in which the state update function consisted of a random model of driver behavior represented by a Markov chain and a deterministic vehicle model. This strategy considered multiple objectives such as fuel economy and drivability while reducing the calculation burden. In Du et al. (2016), an EMS based on SDP and the ECMS was proposed. In the off-line part, SDP was used to divide the historical fixed path driving information into multiple sections. The driving condition model of each section was then established using a Markov chain to solve the minimum fuel consumption.
Table 5Summary of exemplary works on DP-based EMSs.
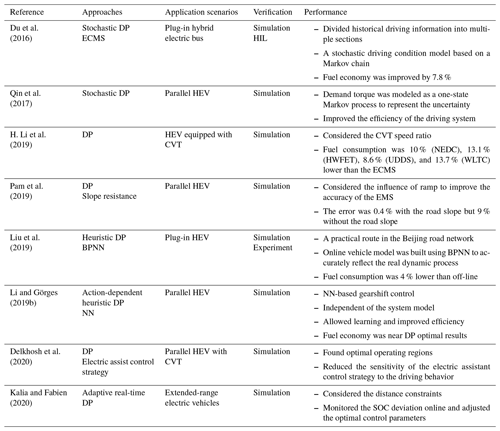
WLTC refers to the Worldwide harmonized Light vehicles Test Cycles.
The characteristics of different DP-based EMSs are illustrated in Table 5. Although the DP method can solve the global optimization solution, it is based on a known travel period. For unknown working conditions, DP obviously cannot meet the actual driving demands of vehicles. The efficiency of energy management is uncertain, so it cannot be directly applied to EMSs and often needs to be combined with other methods.
In addition, the calculation burden of the DP method increases sharply with increases in the system dimension. Using the proposed adaptive DP, heuristic DP, and SDP methods, the traditional DP method combined with various optimization methods can expand the application scope and achieve near-real-time optimization. In addition, according to the characteristics of DP, a variety of acceleration algorithms are also applied to improve the calculation efficiency. At present, the DP-based EMS is mainly used in HEVs with fixed driving conditions, including hybrid electric buses and hybrid electric mine cars. It is often used as a reference to verify the performance of other EMSs.
4.1.2 The PMP-based energy management strategy
Pontryagin's minimum principle is also called Pontryagin's maximum principle. When the state or input is restricted, the optimal control signal from one state to the next state is obtained. The PMP-based EMS mainly achieves global optimization control of HEVs by solving the minimum value of the Hamiltonian. The Hamiltonian function is obtained by combining parameters such as the SOC, fuel consumption, and demanded power with a mathematical model of the HEV, and the optima global solution can be obtained according to the driving conditions (Wu, 2018). A flow chart of the PMP-based EMS is shown in Fig. 5.
In PMP-based EMS, the cost function can be defined as follows:
where ta and tb denote the respective initial moment and the end moment, u denotes the control variable, K(tb) denotes the terminal constraint, L( ) denotes the objective function, and x( ) denotes the boundary condition.
The Hamiltonian function H can be defined as follows:
where λ(t) denotes the co-state variable, and h( ) denotes the state function.
When tb is fixed, for the state quantity of the domain, the state at the initial time and the end time are determined, and the state equation and co-state variables satisfy the following conditions:
For all of the control variables in the domain, the value of H at the optimal control variable is the smallest.
Compared with DP, the PMP-based EMS achieves global optimization with less calculation, which is equivalent to the optimization effect of DP, and is more suitable for real-time control. However, without establishing an accurate real-time predictive model, instantaneous optimization still cannot be achieved.
In Sanchez and Delpra (2018), a PMP-based EMS was proposed to optimize calculation. A Q-trick method was proposed to transform the energy management issue into a boundary value problem and accelerate the operation process. Based on the assumption that the battery's internal resistance and open-circuit voltage are independent of the SOC, instantaneous optimal control with appropriate battery usage equivalent parameters can result in the global optimization solution. According to these findings, a PMP-based EMS was proposed in Kim et al. (2011), who confined the optimal operating line of an ICE under a specific output torque and speed and also determined the appropriate equivalent battery usage parameters. In Yuan et al. (2013), a mathematical expression relating gear shifting to speed was established. A PMP-based EMS was proposed to transform the energy management issue into an optimal control problem based on the cost function. In Li et al. (2015), the fuel consumption, SOC, and battery loss were comprehensively considered for a harsh environment, and the SOC was restricted to a certain range. In addition, a battery operating severity factor was adopted to describe the loss status of the battery, and a PMP-based EMS was then proposed to reduce this factor. In Zhao and Antonio (2016), a PMP-based EMS was proposed and optimized using selective Hamiltonian minimization: a parameter analysis model was used to establish selective Hamiltonian minimization, and the selective Hamiltonian minimization was adopted to select the possible optimal control mode. In Hadj-Said et al. (2017, 2018), considering the discrete variables and continuous variables for PMP-based EMSs, the energy management problem was solved using an analytical method. The power distribution of the ICE and EM, the transmission ratio, and the start–stop process of the ICE were taken as the optimization variables.
Improving the real-time adaptability of PMP-based EMSs has become a hot research issue. In Lee et al. (2019), an adaptive PMP-based EMS established on real-time co-state adjustment according to the current driving conditions was proposed. Among the control process, the key control parameters were updated and balanced adaptively according to the SOC. In X. Li et al. (2019), an adaptive PMP-based EMS established on driving cycle prediction was proposed for fuel cell HEVs. The particle swarm optimization (PSO) algorithm was adopted to classify driving modes, and the Markov model was adopted to predict the speed and driving behavior in different driving modes. In Onori and Tribioli (2015), an adaptive supervisory PMP-based EMS was proposed to achieve online energy management of plug-in HEVs. Its co-state can be adjusted with changes in driving conditions. SOC feedback was used to eliminate the uncertainty in the average speed and total driving distance, and deviation between the actual SOC and the reference linear SOC distribution was prevented by resetting the co-state. The third necessary condition of PMP was only adopted in Nguyen et al. (2018) to derive a closed-form solution containing state variables in order to avoid EMSs relying on additional adaptive mechanisms in real-time control. In Xie et al. (2019), PMP and MPC were combined to realize short-term speed prediction using a Markov chain based on the actual driving cycle, and the EF did not need real-time adjustment; this was done with the aim of improving the calculation efficiency and real-time prediction performance.
The characteristics of different PMP-based EMSs are illustrated in Table 6. The PMP-based EMS improves calculation efficiency, but this method still cannot achieve real-time control. Under the premise of the certain constraint function and EF, the approximate global optimization solution can be obtained according to the vehicle model. The PMP-based EMS needs to work under a known driving cycle and cannot realize online control. Methods based on a predictive model and condition recognition are applied to this EMS. The PMP is used to solve the minimum energy consumption in the prediction domain in order to realize the near-real-time energy management of HEVs.
4.1.3 The GA-based energy management strategy
The GA was initially designed and proposed according to the law of biological evolution in nature. It obtained the optimal solution by simulating the natural selection in Darwin's evolution theory and the biological evolution process in the genetic mechanism. The GA uses mathematical methods and computer simulation to transform the optimization problem into a biology-like evolutionary process. In the GA, the fitness function is adopted to evaluate the merits and shortcomings of individuals. When the fitness of the optimization target reaches a set value, its fitness stops increasing, or the number of iterations reaches a set number, the GA optimization process stops, and the final optimization result is output (Lü et al., 2020). A flow chart of the GA is shown in Fig. 6.
The GA has been widely used in combinatorial optimization and adaptive control because of its simple form, good global optimization performance, and high calculation efficiency. The introduction of the GA provides a new method for solving energy management issues. In the energy management of HEVs, the fuel consumption, emissions, and vehicle performance are generally taken as fitness functions to achieve single- or multi-objective optimization.
where G denotes the fitness; represent the optimized parameters; represent the fitness functions; bi(x) denotes the limiting conditions; and represent the weight of each fitness function.
In Panday and Bansal (2016), different battery models and SOC evaluation methods were adopted to analyze the vehicle performance, and a GA-based EMS was proposed, which can realize the optimization of the start–stop threshold of the ICE and SOC estimation algorithm. In Zhang et al. (2014), a GA-based EMS was proposed to transform the energy management issue into a multi-objective optimization problem including vehicle energy consumption, selected emission species, and the SOC. In addition, the variable domain method was adopted to transform the multi-objective problem into a nonlinear programming problem, which was solved using the GA. In Chen et al. (2014), an EMS combining the GA and quadratic programming (QP) was proposed, which simulated the relationship between the battery current and the fuel efficiency. The GA was adopted to search and optimize the start–stop threshold of the ICE, and QP was adopted to obtain the optimal battery current when the ICE was working. In T. Liu et al. (2018a), a GA-based EMS combined with condition recognition was proposed. The representative operating conditions of the four driving modes were obtained by classifying the historical driving data. The GA was adopted to solve and save the optimal control under different driving modes. The driving mode can be identified online, and the corresponding optimal control can be activated. In Zhan et al. (2016), an EMS based on the GA and the k-means clustering algorithm was proposed. First, four conventional driving modes were selected to obtain the relationship between the equivalent fuel coefficient and fuel consumption. The GA and k-means clustering algorithms were used to identify the driving modes, and the power distribution of the ICE and EM was adjusted in real time.
The characteristics of different GA-based EMSs are illustrated in Table 7. The GA provides a new idea for EMSs, allowing researchers to use the perspective of the vehicle itself. In addition, the GA also has strong adaptability and self-learning habit, which can produce a group of candidate solutions, deal with multiple individuals in the population, and search for various solutions in the space for comprehensive evaluation. However, for a GA-based EMS, there is no definite evaluation method with respect to its accuracy. In the calculation process, the GA is prone to premature convergence, which may affect the final result of the global optimization.
4.1.4 The GT-based energy management strategy
GT mainly studies the interaction between the formulaic incentive structures and is a mathematical theory and method to study competitive phenomena. GT considers the predicted behavior and actual behavior of individuals and studies their optimization strategies. At first, GT was used in economic activities. With the continuous progress of technology, GT has been gradually applied to military science, computer science, and other disciplines. The elements of GT are generally players, strategies, and gains and losses. We assume that the subject of the decision is entirely rational and aims to maximize their interests (Yin and Tian, 2010). GT can be described as an array, including the player Z, the strategies mi, and the cost function Ji. The aim is to identify a range of strategies to satisfy the following:
For HEVs, the players are the ICE, EM, and other power sources, and the common goal is to achieve the best fuel economy and power distribution. In addition, different power sources also have their own revenue goals. The propose of the ICE is to work in the high-efficiency range while reducing emissions, and the propose of the EM is to keep the SOC within an appropriate range.
In Yin et al. (2016), a GT-based EMS was proposed for the hybrid power system composed of an ICE generator, a battery, and an ultracapacitor. Each respective part was taken as the player of GT, and energy management was realized while considering fuel consumption, battery protection, and charge–discharge capacity. Based on Yin et al. (2016), an adjustment program was added in Yin et al. (2018) that could adaptively adjust the weight of each part of the utility functions according to the output of each power source in order to improve the adaptability and real-time performance. In Dextreit and Kolmanovsky (2014), a GT-based EMS was proposed, taking the driver's demand and the power system as two players, where cost penalizing referred to fuel consumption, NOx emissions, SOC deviation, and vehicle running state deviation. The weight of the above parameters can also be adjusted appropriately. In Chen et al. (2015), an adaptive GT-based EMS was proposed to adapt to actual driving behavior. In this strategy, driving modes were predefined according to historical driving data. Each predefined driving mode had its corresponding probability distribution function. Different driving modes adopted different GT strategies to improve adaptability. In J. Xu et al. (2019), a GT-based EMS was proposed containing speed prediction trained using a recurrent NN (RNN) long short-term memory (LSTM) system based on 18 driving cycles. The speed prediction problem was selected to be treated as a multi-series problem to improve the accuracy. In Xu et al. (2021), a GT-based EMS established on prediction was proposed. Speed prediction was realized using a LSTM network. The feature extraction and time series analysis were adopted to improve accuracy. The prediction information was applied to a GT-based EMS to optimize the utility function of different power sources.
The characteristics of different GT-based EMSs are illustrated in Table 8. GT-based EMSs can guarantee good global optimization performance and better consider the performance requirements of each power source. Under the premise of no dependence on driving cycles or driving conditions, it can realize energy management and has strong robustness. In addition, the prediction module and real-time control module can also be added into GT-based EMSs to improve adaptability.
4.1.5 The CO-based energy management strategy
CO is a branch of the field of mathematical optimization, and the objective function is a convex function. Because the local optimization value and the global optimization value of CO are consistent, CO is simpler than the general mathematical optimization process in some aspects (Boyd and Vandenberghe, 2006).
The CO problem can be written as follows:
where γ denotes the convex set, pi(x) denotes the convex function, qj(x) denotes the affine functions, and p0(x) denotes the objective function.
CO can transform the energy management problem of HEVs (such as the gear shifting, the start–stop process of the ICE, the charge–discharge of the battery, the power distribution, and other problems) into a semi-convex definite problem for solving, which significantly simplifies the calculation process while ensuring global optimization performance.
In Song et al. (2017), a CO-based EMS was proposed for a hybrid storage system. A linear approximate model of the composite hybrid storage system was established. The optimal parameters of each power source and corresponding EMS were solved using the CO method with the battery life and the cost of the hybrid storage system as the objectives. In Hadj-Said et al. (2016), a CO-based EMS combining the ICE on/off strategy was proposed. PMP was adopted to optimize the ICE on/off strategy to eliminate its non-convexity, and the corresponding analytical solution was obtained. The analytic solution was integrated into the EMS, and the torque distribution of the ICE and EM was solved using CO. In X. Hu et al. (2013), a CO-based EMS combining the CD and CS strategies was proposed for a plug-in hybrid electric bus. The torque of the EM, the SOC, the output power of the battery, and the ICE generator were set as the optimization objectives. In X. Hu et al. (2016), a CO-based EMS was proposed to optimize the emission characteristics of plug-in HEVs. This strategy can balance the battery output, the charging process, and ICE interactions. The goal of this EMS was to minimize the total amount of CO2 emissions each day and to forecast and update the control process for the next day. In Nüesch et al. (2014b), a DP-optimized CO-based EMS was proposed that considered the influence of frequent ICE starting and stopping as well as frequent gear shifting. According to the driving cycle data, the optimal ICE on/off strategy and the gear-shifting strategy were solved by DP, which were transformed into a convex model. The energy management was then realized using CO. A novel heuristic method was proposed for optimal control, and an EMS was established based on CO and PMP in Murgovski et al. (2013). Only the start–stop process of the ICE was defined as an integer variable, and CO was used to solve the global optimization control. In Freudiger et al. (2020), a CO-based EMS was proposed for a hybrid storage system; this EMS took the power distribution as a decision variable, and CO was applied to reduce the total power loss. In Xiao et al. (2018), a CO-based EMS optimized using a simulated annealing algorithm was proposed. According to the speed and power demand, a convex function based on the fuel consumption of the ICE and battery power was established, and the battery power was controlled by CO.
The characteristics of different CO-based EMSs are illustrated in Table 9. The application of the CO method dramatically improves the calculation efficiency of EMSs. However, for CO-based EMSs, it is necessary to transform the optimization goal of the energy management issue into an appropriate convex model, and the constraint conditions must also be a convex model. For non-convex models, this method cannot solve the problem. Therefore, there are certain limitations in the application of CO, and it cannot meet the requirements of all energy management issues.
4.2 The instantaneous optimization energy management strategy
In research regarding instantaneous optimization EMSs, the ECMS-based EMS and the MPC-based EMS have been widely used. In addition, EMSs based on intelligent algorithms such as RL and NN have been gradually applied to HEVs. A comparison of these different methods is shown in Table 10, which illustrates the pros and cons of each method. This type of EMS was developed along with research into online control. The purpose of this strategy is to minimize the energy or power consumption at the current instantaneously, so that all parts of the HEV are in the optimal working state. This EMS is not restricted by the environment or driving cycle, and it has strong adaptability to unknown driving conditions, a fast response, and a low computational burden. However, this method cannot guarantee minimum energy consumption or emission during the whole driving cycle and cannot achieve global optimization.
4.2.1 The ECMS-based energy management strategy
The ECMS is an instantaneous optimization EMS with excellent performance in practical engineering applications. Its primary content mainly includes two parts: the first part is equivalent fuel consumption, which refers to the cost function established by converting the energy consumed or generated by the EM into the fuel consumption of the ICE using the EF, and the second part is instantaneous optimization, which takes the cost function as the optimization objective and solves the minimum value by adjusting the working state at each instantaneous moment. Therefore, the main problem of the ECMS is to establish a cost function (Jing, 2020). Parameters including battery health and the emission characteristics of the vehicle are also used as weighted terms in the cost function. This EMS can minimize the instantaneous energy consumption and adjust and optimize the working state and emission characteristics. Moreover, this EMS can be combined with various optimization methods to obtain multiple optimization effects. However, the selection of EFs often needs to be based on experience. The quality of EFs directly affects the adaptation of different working conditions.
In Jing et al. (2019), the SOC, the demand torque, and the EM speed were taken as input, and an ECMS-based EMS was proposed. In addition, the PSO algorithm was adapted to adjust EFs in real time to solve the optimal working point of the ICE and EM in order to realize the optimal energy distribution. In Khodabakhshian et al. (2013), an ECMS-based EMS was proposed in which the cost function included fuel consumption and the compensative electrical power consumption. A double variable function was established based on the SOC and its derivative to calculate the EF in the cost function. In Kommuri et al. (2020), an ECMS-based EMS was proposed for the best behavior assessment of HEVs. The battery aging, the start–stop process of the ICE, charging sustainability, and fuel economy were considered. The cost function was established, and instantaneous optimization was performed according to the working constraints of each component. In Nüesch et al. (2014a), an ECMS-based EMS established on the problem of excessive NOx emissions from heavy-duty HEVs was proposed. This strategy introduced NOx emissions into a cost function and tracked a given SOC reference trajectory in real time. In Qiao et al. (2019), an ECMS-based EMS was proposed for NOx and particulate emissions that considered both fuel economy and emission characteristics. The cost function based on fuel economy and different pollutant emissions was established, and EFs of different pollutant emissions' influence on the cost function and optimization process were discussed.
With deepening research, a problem with the adaptability of the EF has been exposed. The fixed EFs cannot fully exploit the energy-saving advantages of HEVs. In order to adjust the EFs, an adaptive ECMS-based (A-ECMS) method has been proposed that can adjust control parameters according to current and future situation requirements. Its basic principle is to adjust the EF in real time according to the predictive model (Onori and Serrao, 2011). The commonly used methods to improve EMS adaptability include the SOC feedback, driving condition prediction, and speed prediction. Flow charts of these methods are shown in Figs. 7–9.
In Musardo et al. (2005), an A-ECMS-based EMS was proposed that combined the current and predicted speed and GPS data to establish the current driving condition. The EF was updated to realize the adaptive EMS. In addition, the effect of the updating frequency of the EF on the adaptability was also considered. In H. Liu et al. (2018b), an A-ECMS-based EMS was proposed based on target driving cycle generation for HEVs under fixed driving conditions. The co-state equation based on PMP was established, and the optimal solution under different initial SOC conditions was obtained. The adaptive cost function, composed of the fixed term and the dynamic term, was designed. The initial value of the fixed term was solved by the interpolation mapping of the initial SOC data and driving data, and the dynamic term was solved by PI (proportional integral) control according to the piecewise SOC reference curve. In Zhou et al. (2021), an A-ECMS-based EMS that integrated ramp information and the mass prediction was proposed. Combined with GPS data, the road slope was estimated, and the vehicle mass was estimated using the recursive least squares method. In addition, the reference trajectories of the SOC under different load conditions were established, and the traditional A-ECMS algorithm was used to track the reference SOC trajectories. In Li and Jiao (2019), an A-ECMS-based EMS established on traffic information recognition was proposed. The k-means clustering algorithm was adopted to divide the historical traffic data into four conditions. According to the current traffic conditions and the SOC, the EF corresponding to each typical traffic condition was solved. In Lei et al. (2020), an A-ECMS-based EMS considering traffic information was proposed. The GA solved the initial EF under different initial SOC conditions, and DP solved the optimal SOC trajectory. Fuzzy logic was used to adjust the EF in real time in order to track the optimal SOC trajectory. An ECMS was used to realize the optimal control. In P. Zhang et al. (2020), an A-ECMS-based EMS established on driving condition recognition was proposed. The driving conditions of heavy-duty HEVs were divided into six categories, and a driving condition recognition method based on NNs was proposed. The EF, the scale factor of a penalty function, and the start speed of the ICE were optimized using the PSO algorithm under each driving condition.
In addition, the combination of an ECMS-based EMSs and rule-based EMSs is very close. EMS control parameters are often optimized by rules, and the EF of the ECMS is adjusted within a certain range. In Vafaeipour et al. (2019), an EMS based on rules and the ECMS was proposed. The driving modes of HEVs were divided into five types using the “if else then” rule. The instantaneous power distribution was defined as a function of the SOC by the ECMS. In addition, the calculation results of the ECMS could also be used as the basis for the division of driving modes. In Li et al. (2017), the rule-based method was used to divide the driving modes, and an ECMS-based EMS was adopted to optimize the energy management issue under hybrid driving conditions. In addition, PSO was used to solve the EF in real time in order to optimize the ECMS-based EMS. In Guercioni et al. (2020), an ECMS-based EMS combining a rule-based gear-shifting strategy was proposed. The optimal gear-shifting rule was obtained using DP, and the gear shifting was carried out according to the rule in the EMS. The torque distribution coefficient of the ICE and EM was optimized in real time. In S. Wang et al. (2019b), fuzzy control was combined with an ECMS-based EMS, and driver intention recognition was introduced into the EMS. Fuzzy control was used to adjust the EF according to the difference between the reference SOC and the actual SOC in order to obtain the optimal charge–discharge trajectory and ensure the continuity of the SOC. In Li et al. (2021), an online SOC estimation method based on a fuzzy inference system (FIS) and an adaptive updated traffic recognition method were integrated into an ECMS-based EMS. FIS was built by an adaptive neuro-FIS (ANFIS) trained by historical traffic data. The adaptively updated traffic recognition method and the estimated SOC value were applied to adaptively adjust the EF. In Liu and Zhang (2017), an ECMS-based EMS established on fuzzy logic driver behavior recognition was proposed. The fuzzy logic was used to identify different driver behaviors, and the EF was adjusted in real time according to the road information. The flow chart of this EMS is shown in Fig. 10. In F. Zhang et al. (2016), the difference between the reference SOC and its actual value as well as the derivative were the input, and fuzzy PI control was adopted to adjust the EF, which was applied to an ECMS-based EMS to improve the robustness, the SOC maintainable performance, and the fuel economy.
With the continuous development of computer science, many intelligent algorithms have been proposed and applied to ECMS-based EMSs; these algorithms are used to achieve adaptive adjustment of EFs and optimize the performance of EMSs. In Wang et al. (2017b), an ECMS-based EMS established on PSO was proposed. The starting speed limit of the ICE was introduced, and the PSO algorithm was used to optimize the EF and the start speed threshold of the ICE under specific driving conditions. In Du et al. (2021), an ECMS-based EMS optimized using the PSO algorithm was proposed; this EMS added a penalty function and modified cost function according to the current SOC. In addition, the PSO algorithm was used to optimize the EF and penalty function of the battery charge–discharge. In Jing et al. (2019), an ECMS-based EMS established on the ant colony algorithm was proposed. The ant colony algorithm optimized the charge–discharge EF in ECMS to obtain the optimal charge–discharge EF off-line. In Li et al. (2018), the driving cycle identification of the k-means clustering algorithm was integrated into an ECMS-based EMS optimized using the GA. Characteristic parameters were extracted based on historical driving data, and driving conditions were divided into four categories. The relationship between different equivalent fuel coefficients and fuel consumption under four typical driving conditions was obtained using an ECMS to obtain the corresponding optimal power distribution. Based on KGA-means, the current driving condition was recognized. In Han et al. (2018), an ECMS strategy based on energy prediction was proposed. Energy prediction was estimated by the prediction velocity calculated by the chain NNs in different time layers. A novel adaptive rule has been developed by eliminating the need to reset the initial EF based on the energy prediction in order to adjust the EF in real time. In Tian et al. (2020), an ANFIS-optimized ECMS-based EMS was proposed considering the regularity and fixity of HEV driving conditions. The optimal control trajectory was obtained using DP, and a set of optimal EFs was obtained using the rolling optimization method, which was used to train an ANFIS. By using a trained ANFIS in the ECMS, the EF of the application was derived, and the online optimal power distribution was then realized.
Table 11Summary of exemplary works on ECMS-based EMSs
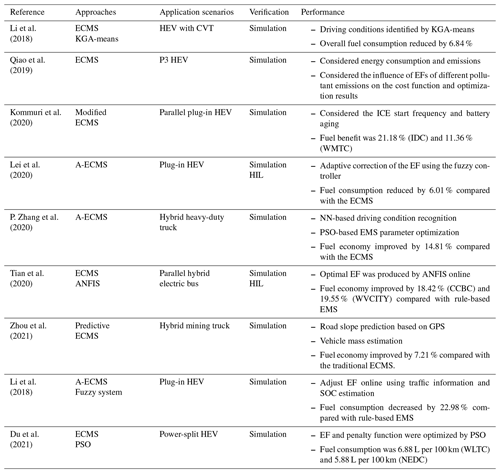
IDC refers to the Indian Driving Cycle. WMTC refers to the World Motorcycle Test Cycle. CCBC refers to the Chinese typical City Bus drive Cycle. WVCITY refers to the West Virginia City Driving Schedule.
The characteristics of different ECMS-based EMSs are illustrated in Table 11. Research regarding ECMS-based EMSs has experienced a transition from fixed EFs to variable EFs. The selection of the EF directly affects the optimal control performance of the EMS. The fixed EF was obtained according to experience or engineering data, representing all driving conditions. With the development of technology, more and more scholars have began paying attention to the adaptability of the EF and have began using different methods to describe the relationship between the EF and different driving conditions. Various intelligent algorithms and predictive models have also been introduced into EMSs to improve adaptability.
4.2.2 The MPC-based energy management strategy
MPC was a new control method. The basic principle of MPC is as follows: at each sampling moment, a finite domain optimization problem is solved according to the current information, and the obtained control sequence is applied to the controlled object. This process is repeated at each sampling moment, and the solution process of the optimization problem is constantly updated with new measured values (Huang et al., 2017). The four characteristics of MPC are as follows: the predictive model, the reference trajectory, rolling optimization, and feedback correction (F. Zhang et al., 2019). The principle and characteristics of MPC are shown in Fig. 11.
The MPC-based EMS transforms the optimization problem of the global condition into a local optimization problem in each predicted time domain. The rolling optimization continuously updates the driving state in the next predicted time domain. Because MPC has strong robustness and high stability as well as integrating rolling optimization and feedback correction, it is applicable to solve the HEV energy management problem with nonlinear multiple degrees of freedom (Morari and Baric, 2006).
In B. Zhang et al. (2019), an MPC-based EMS was proposed to achieve optimal power distribution and fuel consumption minimization. The driving modes of HEVs were selected using rules, and the optimization problem was solved using the sequential QP (SQP) method. In Chen et al. (2021), the demand power of HEVs was divided into high and low frequency, and an MPC-based EMS in the low-frequency band was proposed. The low-frequency power demand was taken as input, and the fuel economy, the SOC, and the busbar voltage were used as optimization targets. In Borhan et al. (2012), the energy management problem was first transformed into a problem considering the linear time-varying cost function. A quadratic cost function considering fuel consumption was then introduced into it, and an MPC-based EMS was subsequently proposed. The second cost function divided the fuel consumption into a stage cost and an approximation of “cost to go” as a function of the SOC. In Zhang and Shen (2016), the power distribution decision was regarded as a sublinear rolling optimization problem, and an MPC-based EMS was proposed. In addition, an online iterative algorithm based on a continuation/generalized minimum residual algorithm was adopted to solve the optimization problem. In C. Xiang et al. (2017a), two MPC methods were adopted to build an EMS according to the length of the sampling time. For a long sampling time, nonlinear MPC was adopted to ensure the ideal SOC state and prevent significant ICE fluctuation. For a short sampling time, linear MPC was adopted to solve the optimization problem composed of the driving demands. In addition, the adaptive Markov chain was adopted to predict the load demand. In Cheng and Chen (2019), an MPC-based EMS was proposed to improve battery aging while also ensuring fuel economy. The fuel consumption, the SOC, and the battery aging index were predicted, and the cost optimization problem related to the above parameters was solved. In Luo et al. (2015), a multi-objective optimization control system was proposed for a new type of intelligent HEV, which used MPC to improve vehicle safety, fuel economy, and comfort; multistep DP was also used to solve the MPC off-line. In Zhou et al. (2017), a kind of MPC-based EMS was proposed for a hybrid storage system composed of a lithium battery and supercapacitor; this EMS could ensure that the system ran within the specified range and reduced the Ah throughput of the lithium battery. In this EMS, the predictive model was updated online, the model status was monitored in real time, and the QP method was used to obtain the optimal control in each solution interval. In Guo et al. (2017), an MPC-based EMS was proposed to divide the energy management problem into the optimal velocity trajectory and the torque distribution solution. The Krylov subspace method was used to solve the maximum velocity trajectory and improve the calculation efficiency. PMP and the numerical method were used to solve the optimal torque distribution and the gear-shifting rule.
Although MPC has a certain degree of robustness in solving uncertain problems, the method has certain limitations due to the fixed function. Scholars have proposed the stochastic MPC (SMPC) to solve these limitations, which included the probability of uncertainty in the optimization problem (Mesbah, 2016). In Qian et al. (2018), an SMPC-based EMS for four-wheel drive (4WD) HEVs was proposed. A Markov predictive model was applied to predict the acceleration and solve the demand torque. The rolling solution was carried out using DP to achieve the optimal control of fuel economy. In Xie et al. (2017), an SMPC-based EMS with a variable predictive time step was proposed to prevent the practical application interruption caused by driving state defects. A Markov predictive model was used to predict the velocity series, and average filtering and quadratic fitting were adopted to reduce the fluctuation in the predicted results. Online estimation and a variable threshold were adopted to predict the time variation in the time step, and SMPC was then used to solve the instantaneous optimal. In Cairano et al. (2014), an SMPC-based EMS was proposed combined with driver behavior learning based on the Markov model and a scenario-based approach for stochastic optimization and QP. According to the constraints of the SOC and battery charge and discharge power, SMPC was used to solve the optimal power distribution between the battery and the engine. In Li et al. (2016), an SMPC-based EMS based on “drive behavior aware” was proposed. The k-means algorithm was used to classify driver behaviors, and Markov models under different behaviors were established. When driver behaviors were regarded as random disturbances, an SMPC-based EMS optimized by the ECMS was used to eliminate some worsened fuel economy work points.
Scholars have added an intelligent algorithm-based speed prediction model, a road information prediction model, a driving condition prediction model, and q driver behavior prediction model to the traditional MPC-based EMSs to optimize their performance. In C. Xiang et al. (2017b), an EMS based on a nonlinear MPC (NMPC) was proposed that adopted a slow sampling time to maintain the SOC. In addition, a radial basis function NN was used to predict the short-term speed, and the optimal problem was solved using DP. In C. Sun et al. (2015a), the prediction accuracy, calculation cost, and fuel economy of an MPC-based EMS established on exponential variation was proposed. A random Markov chain as well as speed prediction based on NNs were evaluated to optimize the EMS. In Guo et al. (2019a), an adaptive MPC-based EMS was proposed. The SOC reference constraint of each MPC calculation step was obtained by solving the dynamic traffic information of the target driving task. In addition, the speed prediction model based on NN was used for short-term speed prediction, and the optimal energy allocation in the prediction domain was solved using DP within the framework of MPC. In Yang et al. (2020), an MPC-based EMS considering road ramp was proposed. The optimal speed was calculated based on the prediction of ramp information, and the power distribution and gear shifting based on MPC were then optimized based on the calculated speed. In Guo et al. (2019b), an MPC-based EMS established on ramp and speed prediction was proposed. A data-driven autoregressive integrated moving average model was applied to predict the future speed and road ramp of HEVs in real time in order to provide a reference for the speed and road ramp for the EMS, and this was used as input to solve the driving demands. In C. Sun et al. (2015b), an MPC-based EMS established on real-time traffic data prediction was proposed. The traffic prediction information could be used to establish the average speed of the current section and solve the SOC reference trajectory, which could be applied as the constraint condition of the SOC. In Park and Ahn (2019), an MPC-based EMS established on future driving cycle prediction was proposed. The radar signal and vehicle operation signal were used as input, the future driving cycle was predicted by deep NN, and the predicted near-future driving cycle was used as input to solve the optimal energy distribution.
Table 12Summary of the exemplary works on MPC-based EMS.
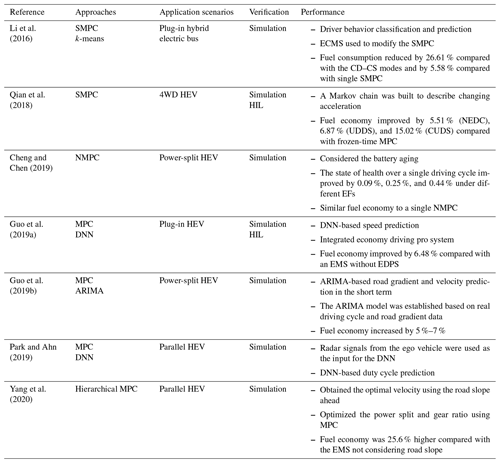
CUDS refers to the China Urban Driving Schedule. EDPS refers to the Economy Driving Pro System.
The characteristics of different MPC-based EMSs are illustrated in Table 12. MPC-based EMSs can efficiently solve the optimization problems with multivariable constraints and can also overcome the uncertainty of the system to a certain extent, due to strong robustness and stability. The introduction of SMPC can avoid the influence of random constraints to a large extent and further improve the adaptability of the control system. In addition, a variety of prediction-based methods have been introduced into EMSs and can improve their adaptability to unknown driving conditions. However, multiple optimization goals often need to be considered. The mutual restriction and influence of various performance indicators will inevitably introduce more constraints. It is still a difficult point for intelligent algorithms to optimize the calculation process and ensure real-time performance. In addition, in the complex road environment, the randomness of human–vehicle–road interaction will also affect the control performance of MPC.
4.2.3 The RL-based energy management strategy
RL is a method used to describe and solve an agent's interaction process with the environment in order to achieve the maximum return or a specific goal. It has been developed from animal learning and parameter disturbance adaptive control theory and is currently applied in automatic control. RL uses a framework to define the interaction between the agent and the environment in terms of state, action, and reward (Sutton and Barto, 1998).
RL has obvious advantages in dealing with uncertainty problems and can realize the optimization process through training. In HEV energy management problems, action is generally defined as control variables in EMSs, such as the speed, the torque of the ICE and EM, and other parameters. The state is the real-time working state of all parts of the HEV in operation, such as the speed, the SOC, and the current driving conditions. The reward function is the final control goal that needs to be achieved, including the fuel economy, the SOC stability, the battery health, and the emission characteristics (X. Hu et al., 2019). A schematic diagram of an RL-based EMS is shown in Fig. 12.
In Zou et al. (2016), an RL-based EMS for a hybrid tracked vehicle was proposed, and a control-oriented hybrid tracked vehicle model and a power demand model were established. A recursive learning algorithm for power demand transfer probability matrices (TPMs) established on the Markov chain was proposed based on historical data. The Kullback–Leibler divergence rate was applied to measure the difference in power demand TPMs. When the difference was significant, the RL-based EMS was updated in real time. In Xiong et al. (2018), aiming at the optimal power distribution problem between the battery and supercapacitor in a hybrid storage system, an RL-based EMS that judged and updated according to the Kullback–Leibler divergence rate was proposed. The power TPM was solved using long-driving-period data. A control strategy based on a reverse learning algorithm was established, and the TPM was updated according to the applied driving cycle. In Sun et al. (2020), according to the ECMS principle, a data-driven RL-based EMS was proposed. An optimization problem with the SOC retention and fuel consumption as objectives was established. The EMS was simplified by an adaptive fuzzy filter. In Liu et al. (2020), a double-layer adaptive RL-based EMS was proposed. The upper layer calculated the corresponding TPMs according to the drive cycle change, and the induced matrix norm was adopted as the standard to identify the transformation. The lower layer was based on the model-free RL and used the transformed TPMs to solve the optimal power distribution. In Hu et al. (2018), an EMS based on deep RL (DRL) was proposed for adaptability to different driving conditions. The reward function was directly related to fuel consumption. The EMS adopted the QNN (Quantized Neural Networks) online learning method to obtain the action from the state and to realize the identification and conversion of driving conditions. In order to solve the problem of the large control variable space in the EMS, the battery performance and the optimal brake specific fuel consumption curve of the HEV were introduced into the DRL-based EMS in Lian et al. (2020). The state parameters and optimization parameters based on the HEV itself can ensure training accuracy. In B. Xu et al. (2019), an RL-based EMS established on Q-learning was proposed. The EMS took the speed and demand torque as the state of Q-learning, chose the demand torque of the EM as the action, and the reward function included the fuel consumption of the ICE and the equivalent battery fuel consumption. During the actual driving process, the Q-value table would be updated adaptively every time. In T. Liu et al. (2018b), an RL-based EMS established on speedy Q-learning was proposed, which adopted the real-time learning model to calculate the TPMs of power demand under different driving conditions and also distinguished the TPMs using the induction matrix norm. In addition, the speedy Q-learning algorithm was adopted to accelerate the calculation convergence speed of the Markov chain. In Han et al. (2019), a double deep Q-learning-based EMS was proposed to optimize the energy management problem while maintaining the stability of the SOC. In addition, double deep Q-learning was used to correct the error caused by the overestimation of the cost function. In Xu et al. (2020), it was proposed that the effectiveness of an RL-based EMS largely depended on the selection of optimization parameters. Thus, this paper explored the aspect of EMSs caused by the number and selection of states, the discretization of states and actions, and the selection of the learning experience. The results showed that increasing the discretization of states will decrease the fuel economy, whereas increasing the discretization of actions will increase the fuel economy.
Table 13Summary of exemplary works on RL-based EMSs.
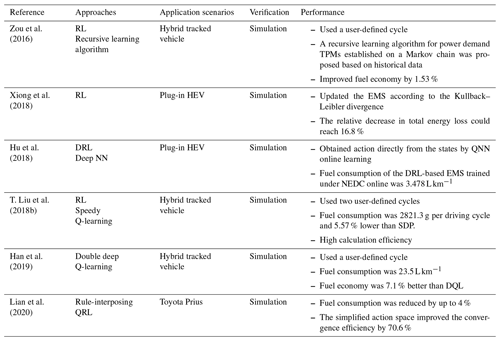
QRL denotes Q-reinforcement learning. DQL denotes deep Q-learning.
The characteristics of different RL-based EMSs are illustrated in Table 13. As a model-free control method, RL-based EMSs have been widely used in HEV energy management in recent years due to their good adaptability to different driving conditions and high calculation efficiency. However, there are still major problems with selecting parameters for this type of EMS, and the effect of different parameters on the RL framework is still unknown. In addition, RL needs to be trained according to a large amount of data and constantly updated in the application. Although RL can achieve instantaneous optimization, its optimization performance is not as excellent as other EMSs. Therefore, it is necessary to add constraints based on vehicle information into the RL framework to carry out optimization.
4.2.4 The NN-based energy management strategy
An NN is an adaptive information processing system based on the interconnection of many processing nodes. Models of the human brain are built from information processing, and networks are formed according to certain connections. The NN is composed of many connected nodes, and each node represents an activation function. An NN has strong self-adaptability and self-learning functions that can learn and update in real time according to changes in the system (Dougherty, 1995). NNs are generally divided into an input layer, a hidden layer, and an output layer. The topology and weights determine the performance of an NN. The topological structure ensures strong nonlinearity. The weights need to be trained by NNs based on large amounts of data. In addition, the NN also has the function of back-propagation, which can reduce the final output error by comparing the output value with the target value to solve the error and reverse input (Baumann et al., 1998).
In NN-based EMSs, parameters such as the current vehicle speed, the SOC, output information from the EM and ICE, and demand torque are generally taken as the input layer. In contrast, the output layer is generally the optimization target, including the optimal power distribution, fuel consumption, equivalent fuel consumption, and emissions of the HEV. In addition, this type of EMS is trained based on a large number of historical data collected under various driving conditions. The network is constantly updated and optimized for practical use. The control structure diagram of an NN-based EMS is shown in Fig. 13.
Table 14Summary of exemplary works on the NN-based EMS
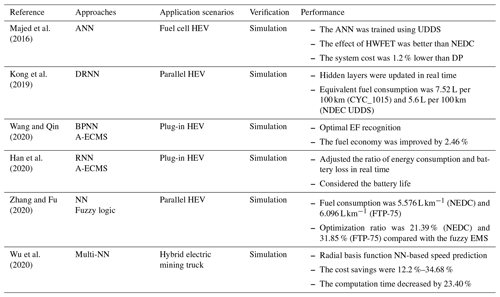
CYC_1015 represents the Japanese 10–15 mode driving cycle. NDEC represents the New European Driving Cycle.
In Tian (2018), an NN-based EMS was proposed for a hybrid electric bus under fixed driving conditions that took the demand torque, speed, SOC, and mileage ratio as input and obtained the optimal power output of the ICE. Due to the continuous accumulation of determined conditions, the obtained optimal data set for NN training was also increased. In Majed et al. (2016), an NN-based EMS was proposed for a fuel cell HEV. In this strategy, the driving demands of the HEV were firstly predicted, and the predicted results, the SOC, and the fuel cell power were taken as the inputs for the NN. In addition, the optimal results obtained by DP were used for off-line training of the NN. In Q. Xu et al. (2018b), an NN-based EMS was proposed based on the principle of minimum power loss. This strategy took the demand torque, speed, and SOC as the input layer, and the output power of the ICE and the speed were obtained after calculating the hidden layers. Considering the efficiency of the transmission system of a dual-mode HEV, an NN-based EMS was proposed in Qi et al. (2015). The GA was applied to train the weights of the NN. Based on the transmission efficiency model, the optimal control strategy of the ICE speed was established and integrated into the EMS. In Ates et al. (2010), an NN-based EMS combined with a wavelet transform was proposed for a fuel cell/ultracapacitor hybrid storage system. Wavelet transform was used to reduce the power fluctuations in fuel cells and keep them in a steady state, while NNs were used to control the charge depletion of the ultracapacitor. In Wang and Qin (2020), the ECMS strategy was integrated into the BPNN-based EMS. The EF was used as the intermediate variable to solve the global optimization sequence, and BPNN was then used to extract the optimal sequence and form an online EMS. In Han et al. (2020), an RNN-based EMS was proposed. This strategy could adjust the ratio of energy consumption and battery loss in the EMS in real time. The SOC trajectory was calculated using DP for training. It could also control the deviation between the training value and the actual value in a practical application. In Kong et al. (2019), an EMS based on deep RNN (DRNN) was proposed. Multiple parameters related to the current driving condition of the HEV were selected as inputs, and the optimal output torque of the ICE was obtained through the hidden layers. In addition, the weights in the hidden layers were updated in real time. In Chen et al. (2020), an EMS based on convolutional NN (CNN) was proposed for condition identification. This strategy divided the conventional driving conditions into six types using the K-shape clustering method, and the CNN was trained based on the initial driving cycle and forecasted the driving conditions. In Zhang and Fu (2020), an EMS based on fuzzy logic and an NN was proposed to improve the adaptability of EMSs to different driving conditions. The EMS realized the identification of driving cycles through the sample learning and feature extraction of the NN and used it as the input for the fuzzy logic controller to optimize the MF. In Wu et al. (2020), an EMS based on multi-NNs was proposed. The global optimization results were obtained using DP, the condition recognition NN was trained by the calculation results, and the online co-state estimation was realized using RNN, which was used as the initial value of battery power optimization.
The characteristics of different NN-based EMSs are illustrated in Table 14. The NN-based EMS is a model-free control strategy with a strong learning ability that can deal with the nonlinear optimal energy management problem in real time. It can optimize and update the control process to realize the self-adaptation of the algorithm itself. However, it is difficult to explain the reasoning process of an NN, and the calculation cost is high. In addition, in the case of insufficient data, the NN-based EMS finds it difficult to achieve the optimization effect. Therefore, an NN is often used in combination with other algorithms to form a multi-method control system, which integrates the self-learning performance of an NN into the adaptive EMS to obtain better adaptability.
The ITS refers to the transportation environment in which vehicles, infrastructure, and portable devices are interconnected. It effectively applies information technology and sensor technology in transportation to strengthen the connection between vehicles, roads, and people, thereby forming a real-time, efficient, and accurate transportation system. However, the main problems faced by the ITS with respect to its development include comprehensive access to the traffic status, an accurate understanding of vehicle running conditions and the behavior of other traffic participants, and the provision of more effective traffic information according to vehicle traffic conditions and the related states of mutual interaction (Peng, 2019). The architecture of the ITS is shown in Fig. 14.
The introduction of “Internet of Vehicles” (IoV) technology can solve these problems to a large extent. IoV refers to the system network of data exchange between vehicles, roads, and people based on the internal network, inter-vehicle network, and onboard mobile network through radio frequency identification (RFID) technology, which can realize the integrated control of traffic management and vehicles (Yang et al., 2014). The architecture of IoV is shown in Fig. 15.
With the continuous development of the ITS and IoV technology, the application of intelligent traffic data information to the optimization control of HEV energy management has become a hot topic for scholars (Gu et al., 2019). Generally speaking, ITS-based EMSs use two methods: the first method is based on using static data (e.g., navigation information and historical traffic data) to predict driving conditions in the predicted time domain to realize energy management, whereas the second method is to monitor and predict the behavior of nearby vehicles, traffic signals, and traffic participants according to the dynamic real-time traffic information provided by IoV in order to find the optimal speed to achieve energy management.
5.1 ITS-based EMSs established using static data
ITS-based EMSs established using static traffic data usually classify different driving condition data based on vehicle historical driving data, navigation information and GIS information, and they use an adaptive algorithm to predict the future driving state in the time domain, thereby realizing energy management in the prediction domain. This method mainly adopts the idea of hierarchical control. The upper layer is the classification and prediction layer, which classifies the driving conditions of vehicles according to the historical data and also predicts the driving parameters and driving conditions of the current vehicles. The lower layer adopts the corresponding EMS according to the prediction results to realize the optimal energy allocation of vehicles
In Wei et al. (2016), driving pattern recognition (DPR) was introduced into the ITS-based EMS. The fuzzy rule was designed based on the DPR algorithm that determines the current driving mode based on the historical vehicle data in the upper layer, and fuzzy logic was applied to solve the energy management problem. While driving conditions changed frequently, the MF was adjusted according to DPR results. In R. Zhang et al. (2019), multilayer perceptual NNs were used to extract the features of historical driving conditions to realize the DPR, which was introduced into the ITS-based EMS. Under different driving conditions, the optimal power distribution was realized by fuzzy control. In addition, the GA was used to optimize the critical parameters in the MF, which can reduce the current fluctuation under various driving conditions. In Zhang and Xiong (2015), an ITS-based EMS established on DPR was proposed that used fuzzy logic to divide and identify current driving modes according to the historical information from the last 100 s. An optimal EMS was then developed using DP in order to realize real-time adaptive energy management. In Kim et al. (2019), an ITS-based EMS with DPR was proposed for HEVs with repeated driving conditions, as it can update control parameters in real time by analyzing the past driving modes. The trip information was also updated every day to optimize the DPR. The effective average power and the effective SOC drop rate were selected to determine the driving mode. In the lower layer, PMP was applied to obtain the optimal control results. In C. Wang (2020), an ITS-based EMS was proposed in which the current driving condition was predicted according to the historical driving condition data. According to the predicted driving condition, the GA was applied to solve the energy management issue with the gear-shifting model. The vehicle fuel consumption was taken as the fitness function to avoid the influence of multiple EFs. In Rios-Torres et al. (2018), the diving conditions of HEVs were divided into volatile, normal, and calm according to historical driving data, and an ITS-based EMS was then proposed. In addition, the driving conditions were estimated using real-time vehicle operating parameters. The current driving condition was determined, and ECMS was applied to reduce fuel consumption. Under calm conditions, fuel consumption was reduced by 13 %. In Sun et al. (2016), an ITS-based EMS established on speed prediction was proposed. The prediction of the future short-term driving condition was realized using NNs speed prediction and historical data. A-ECMS was applied to realize the adaptation of EFs by the prediction results, and the driving behavior was then adjusted. In Haußmann et al. (2019), an ITS-based EMS established on driving behavior recognition was proposed. The learning method was used to classify the historical driving data, and the driving conditions were divided into four categories. LSTM-RNN was used to identify driving behaviors online and select the corresponding optimal driving mode based on driving parameters such as speed, acceleration, and braking distance. The optimal power distribution was obtained using the ECMS. In Lian et al. (2017), an ITS-based EMS combining speed prediction based on identifying driving intention and historical driving speed was proposed. A nonlinear autoregressive NN was used to predict the speed, and the demand torque was then predicted. Following this, a mixed logical dynamical model was established to obtain the optimal power distribution within the predicted speed range. In Wang et al. (2020), an ITS-based EMS driven by a large amount of historical driving data was proposed, and the DRL framework was applied to realize the optimal power distribution trained by the large-scale HEV driving conditions. The reward function was established to minimize fuel consumption and power consumption while maintaining power stability. In H. Liu et al. (2018a), an ITS-based EMS established on radial basis function NN speed prediction was proposed. The ramp information from GIS was applied to plan the SOC trajectory in real time. The predicted speed and SOC trajectory were used as the input for the EMS. In the framework of MPC, the energy management problem was solved, fuel economy was improved, and the SOC was maintained in real time. According to the influence of ramp on EMS, an ITS-based EMS established on slope prediction was proposed in Zeng and Wang (2015). According to the driving condition and terrain information, the predictive model of road ramp based on the Markov chain was established. In the optimal layer, the SMPC and SDP were applied to realize the energy management according to the SOC and the ramp prediction model. In Wu et al. (2008), an ITS-based EMS was proposed combined with the BPNN. The energy management rules obtained by a road condition information off-line simulation were introduced into the EMS. The fuzzy c-means cluster was applied to classify the rules. The rules were used to train the NN in order to obtain the optimal ICE output.
The characteristics of different ITS-based EMSs established using static data are illustrated in Table 15. This type of EMS can realize efficient energy management of vehicles in the prediction domain. It can integrate the characteristics of various driving conditions and combine the advantages of a large number of historical data to train the EMS optimization process with the formation of an approximately optimal control process. In addition, the integration of geographic information and navigation information can improve the prediction performance of the driving path to a certain extent and adjust the EMS according to the actual road condition information to improve the control effect.
However, this EMS is highly dependent on the prediction accuracy, and the prediction effect of different prediction algorithms is also different. When the prediction effect is not close to the actual driving process, the vehicle energy management effect may not be optimal. Therefore, the key to this EMS is to select a suitable prediction method to improve the prediction accuracy.
5.2 The ITS-based EMSs established using real-time data
ITS-based EMSs established using real-time traffic data involve the optimal power distribution of the vehicle driving system and include the autonomous planning of the vehicle running state by the target vehicle according to the specific driving environment. This method also mostly adopts the idea of hierarchical control. In the upper layer, the speed optimization method is put forward according to the signal phase and timing (SPAT) and the vehicle running status information. This is to avoid the vehicle being in the frequent deceleration and stop position and to ensure that the vehicle is in the high-efficiency running speed range (Wang, 2020). In addition, according to the speed optimization results in the lower layer, the corresponding optimization method is used to realize the optimal power allocation, and the fuel economy of the HEV can be improved.
In Qian et al. (2017), an ITS-based EMS was proposed according to the signal light information under the ITS framework; this strategy improved fuel economy by avoiding the HEV having to stop at a red light. SPAT was used to calculate the velocity range of the target, and F-MPC was then used to calculate the optimal velocity sequence at a given time. According to the optimal speed sequence, energy management is realized using the ECMS based on the Willan line method. In Qian et al. (2016), an ITS-based EMS established on vehicle-to-vehicle (V2V) communication and vehicle-to-infrastructure (V2I) communication was proposed. The optimal target velocity was obtained by SPAT, multi-island GA, and NMPC. An A-ECMS was used to determine the optimal energy distribution and obtain the optimal output power of the ICE and EM, which could improve fuel economy while avoiding the shutdown of the HEV due to red lights. In X. Qi et al. (2017), an ITS-based EMS was proposed that divided the optimal speed curve of HEVs passing the signal light into four working conditions according to the SPAT. The appropriate working condition curve was selected according to the actual running state of the vehicle, and the demand power of the vehicle in the running process was predicted by MPC. The CD–CS strategy was used to solve the energy management problem. In F. Zhang et al. (2017), an ITS-based EMS established on future vehicle speed prediction was proposed. According to the real-time traffic information provided by V2V and V2I, a CNN was used to predict the speed in different time ranges, and the ECMS was used to achieve energy management. In addition, the effects of predicted speed on the EF and fuel economy were considered, and the EF was adjusted adaptively. In Gong et al. (2008), historical traffic information and a real-time traffic signal sequence based on the ITS were applied to travel modeling, and an ITS-based EMS was proposed. In addition, GPS-based driving path information and sensor-based real-time traffic information were also integrated to model and predict traffic flow. Following this, in the optimization layer, the DP method was used to realize the power depletion control to fully exploit the fuel-saving advantage of the battery and ensure that the SOC was not lower than the minimum value. In Yang et al. (2016), an ITS-based EMS was proposed stemming from the real-time communication connection between the vehicle and the control center. The driving conditions were classified based on the historical data. Following this, the current driving data were analyzed, and the support vector machine method was used to predict the driving conditions. In addition, energy management was realized by combining the real-time information from the control center and the GIS ramp information. In Yang et al. (2017), an ITS-based EMS was divided into online and off-line parts, and a corresponding cloud computing framework was proposed. In the off-line part, driving conditions were classified, and drivers' driving demands were predicted using the real-time data transmitted to the control center. For the online part, a well-trained support vector machine was used to recognize the driving state and select the corresponding driving behavior. The stochastic receding horizon control method was adopted to obtain optimal energy management. In Li (2016), an ITS-based EMS was proposed that was established on the cruising speed of the vehicle in front. The navigation system collected the speed in front, and the trajectory of the collected cruising speed was optimized under the premise of the safety rules. In the optimization layer, based on the optimized cruising speed and the real-time running state of the HEV, DP and MPC were used to realize energy management, which ensured cruise safety and improved the fuel economy of the HEV. In C. Sun et al. (2015b), an ITS-based predictive EMS established on real-time traffic flow data was proposed. An additional SOC planning layer was constructed based on the real-time traffic data, and the optimal SOC trajectory was solved in real time using DP. The energy management in the prediction domain was realized. Meanwhile, the update time of the SOC trajectory was similar to that of traffic flow information. In J. Hu et al. (2016), an ITS-based EMS was proposed in order to maximize the fuel economy of a HEV driving on an unsmooth road. In addition, the current and future driving information, including the dynamic speed limit and terrain information from GIS, were taken as the input for the controller. The optimization results were solved and accelerated by PMP considering the vehicle speed, the power distribution, and the engine working point. In Liu et al. (2021), an ITS-based EMS was proposed based on IoV and real-time traffic information. The speed limit curve was proposed and transformed into a spatial domain problem. According to the speed value based on driving distance, the speed planning and power distribution of HEVs were realized using DP considering the shift limit and speed fluctuation. A time adjustment factor was added to the cost function to constrain the final driving time. In He et al. (2021), physical and network systems were integrated, and an ITS-based EMS established on a cyber-physical system was proposed. The historical traffic information of a hybrid electric bus and DRL were used to conduct exploratory training for the EMS. The prior effective knowledge of the hybrid electric bus was then applied to a Toyota Prius by deep transfer learning to accelerate the convergence speed of the new EMS. In addition, V2V and V2I were combined to obtain the status parameters of surrounding vehicles and signal lights, and different operation modes were selected under different traffic conditions to realize the optimization of energy management problems.
Table 16Summary of exemplary works on ITS-based EMSs established using static data.
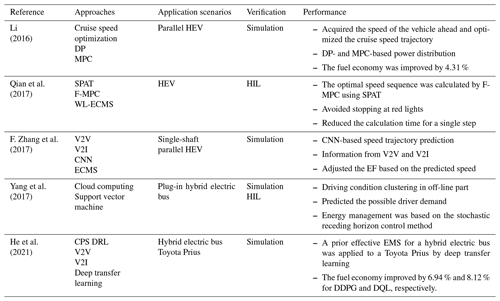
F-MPC represents fast MPC. WL-ECMS represents the Willan-line-based ECMS. CPS represents the cyber-physical system. DDPG represents the deep deterministic policy gradients.
The characteristics of different ITS-based EMSs established using real-time data are illustrated in Table 16. This type of EMS is based on real-time data, which can preset the operating rules and driving condition classification and provide the appropriate future operating state according to the real-time operating state of the vehicle in order to deal with changes in traffic information. Moreover, the behavior of the driver and the running state of the vehicle can be guided. However, this EMS has high requirements for infrastructure and relies on the real-time information interaction between the vehicle and the cloud control center. The prediction interval is also often long (e.g., 100 s). Hence, this EMS does not work well in environments with weak infrastructure or poor communication. With the continuous development and progress of technology, this strategy has a broad application prospect.
In this paper, EMSs of HEVs have been reasonably classified, and the current research results have been summarized. The rule-based EMS can obtain optimization results to a certain extent and has been widely used in practice. However, this EMS cannot achieve optimal fuel economy and fully exploit the energy-saving potential of HEVs. The global optimization-based EMS can reduce fuel consumption to the greatest extent across the entire range of driving conditions. However, it cannot be directly applied to real vehicles and is often used as a benchmark for other EMSs. In contrast, the instantaneous optimization-based EMS considers real-time performance and instantaneous fuel consumption minimization and does not require a priori knowledge of the driving cycle. Hence, it has better fuel economy and adaptability. However, this EMS is not the global optimization solution of the whole driving cycle. In addition, vehicle structural parameters, driving cycles, the determination of the cost function, and driving cycle prediction methods will affect the accuracy and effect of EMSs. Therefore, the improvement and optimization of EMSs is an effective way to balance fuel economy and real-time performance. The characteristics of the different EMSs are summarized in Table 17.
According to the current problems and the development trend of HEVs, four future research directions are proposed and are outlined in the following subsections.
6.1 EMSs based on the transient response of the HEV power system
In existing studies, the EMS is usually used as the upper controller for power distribution. Its optimization target is mainly based on the steady-state index, without taking the transient response of the components of the underlying power system into consideration. The vehicle-integrated controller needs to solve the demand and also needs to collect the vehicle running data to realize the monitoring and diagnosis of the HEV, which has high requirements for the response speed of the control system. However, during the actual driving process, transient conditions such as rapid acceleration, rapid braking, and parking account for about one-third of the driving conditions experienced. These transient response processes will be directly reflected in the power train, and the controller needs to control the bottom actuator according to the rapidly changing driving conditions. The transient response of the bottom actuator has become the key point of optimization. The transient fuel consumption and frequency response characteristics of the ICE will affect the fuel economy. In the calculation, these problems are often reduced or ignored by simplified vehicle models. Therefore, balancing and optimizing the response characteristics and real-time performance of the bottom actuator while meeting the requirements of approximate optimal control and calculation efficiency at the upper level has become a research focus.
6.2 EMS based on multi-objective optimization
Because a HEV has multiple power sources, the influence of interactions among them on performance cannot be ignored. The initial requirement for EMSs was generally the improvement of the fuel economy performance. With the continuous development of technology, scholars have also added the performance of various components of power systems into EMSs and have transformed EMSs from single-objective optimization to multi-objective optimization scenarios. At present, EMSs not only take overall fuel economy into consideration but also consider pollutant emissions, battery degradation, transient fuel consumption, and SOC retention. In addition, EMSs also need to consider the interactions between the power sources and the performance, such as fuel economy and drivability, the SOC charge–discharge characteristics, and the battery life. However, introducing the above factors into EMSs and realizing multi-objective optimization according to their interaction also needs to be paid more attention in future research.
6.3 EMS based on multi-method collaborative optimization
At present, EMSs based on a single method have their disadvantages, such as low calculation efficiency, poor adaptability, and poor real-time performance, which leads to different limitations in the optimization process and makes it difficult to fully exploit the performance potential of HEVs. For example, the fuzzy logic rule-based EMS has good real-time performance, but the optimization effect is limited. While DP can solve the global optimization solution, the driving conditions need to be known in advance, and the calculation burden is heavy. Therefore, in the process of EMS development and design, combining the advantages of various methods to achieve collaborative optimization control will be a continuous research hot spot.
6.4 EMSs based on driving condition and driver behavior prediction
Although many EMSs are established based on driving conditions, the driving conditions of HEVs are unknown during the actual driving process, which makes it impossible to obtain the optimal control results. Therefore, knowing the driving conditions in the future or for a short time is of great help with respect to improving the control performance of EMSs. At present, driving condition prediction methods based on NNs and RL are applied to EMSs, and the application of location information such as GIS and GPS also provides great help for online driving condition prediction for EMSs. In recent years, with the rapid development of the ITS and IoV technology, the vehicle can realize the planning of energy management according to the current position information and known driving condition data. In addition, regarding the subject of driving behavior, the driver will change the driving state according to subjective judgment. Different drivers may take different action in the same situation, which introduces uncertainty with respect to the driving behavior and even with respect to driving conditions. Thus, the realization of driver behavior prediction is also very helpful in predicting driving conditions. However, in the current research, the accuracy of driving condition prediction and driver behavior prediction is still difficult to guarantee, which has become a problem for future development. Therefore, future research will focus on combining the prediction process with EMSs efficiently and stably while also ensuring prediction accuracy.
A-ECMS | Adaptive equivalent consumption minimization strategy |
ANFIS | Adaptive neuro-fuzzy inference system |
BPNN | Back-propagation neural network |
CD | Charge depleting |
CNN | Convolutional neural network |
CO | Convex optimization |
CS | Charge sustaining |
DP | Dynamic programming |
DPR | Driving pattern recognition |
DRL | Deep reinforcement learning |
DRNN | Deep recurrent neural network |
ECMS | Equivalent consumption minimization strategy |
EF | Equivalent factor |
EM | Electric motor |
EMS | Energy management strategy |
EV | Electric vehicle |
FIS | Fuzzy inference system |
GA | Genetic algorithm |
GIS | Geographic information system |
GT | Game theory |
HEV | Hybrid electric vehicle |
ICE | Internal combustion engine |
IoV | Internet of Vehicles |
ITS | Intelligent transportation system |
LFS | Load-following strategy |
LSTM | Long short-term memory |
MF | Membership function |
MPC | Model predictive control |
NMPC | Nonlinear model predictive control |
NN | Neural network |
NOx | Nitrogen oxide |
PFS | Power-following strategy |
PMP | Pontryagin's minimum principle |
PSO | Particle swarm optimization |
QP | Quadratic programming |
RL | Reinforcement learning |
RNN | Recurrent neural network |
SDP | Stochastic dynamic programming |
SFS | Speed-following strategy |
SMPC | Stochastic model predictive control |
SPAT | Signal phase and timing |
SQP | Sequential quadratic programming |
TPM | Transfer probability matrices |
V2I | Vehicle to infrastructure |
V2V | Vehicle to vehicle |
No data sets were used in this article.
XL conceived the study. YZ and SL carried out the literature search. YZ and XL undertook the formal analysis. YZ, QL, and YX drafted the manuscript; YZ, QL, and SL were responsible for writing the final version of the paper; YZ typeset the paper; and XL and YX reviewed and edited the paper. YZ, QL, and SL created the figures. XL and YZ supervised the study. YZ, SL, and YX aided with translation. All authors have read and agreed upon the published version of the paper.
The contact author has declared that neither they nor their co-authors have any competing interests.
Publisher's note: Copernicus Publications remains neutral with regard to jurisdictional claims in published maps and institutional affiliations.
This paper was edited by Daniel Condurache and reviewed by two anonymous referees.
Asghar, M., Bhatti, A. I., Ahmed, Q., and Murtaza, G.: Energy Management Strategy for Atkinson Cycle Engine Based Parallel Hybrid Electric Vehicle, IEEE Access, 6, 28008–28018, https://doi.org/10.1109/ACCESS.2018.2835395, 2018.
Ates, Y., Erdinc, O., Uzunoglu, M., and Vural, B.: Energy management of an FC/UC hybrid vehicular power system using a combined neural network-wavelet transform based strategy, Int. J. Hydrogen. Energ., 35, 774–783, https://doi.org/10.1016/j.ijhydene.2009.11.021, 2010.
Baumann, B., Rizzoni, G., and Washington, G.: Intelligent Control of Hybrid Vehicles Using Neural Networks and Fuzzy Logic, SAE Technical Paper, SAE International, https://doi.org/10.4271/981061, 1998.
Bertsekas, D. P.: Dynamic programming and optimal control, 1st edn., Athena Scientific, Massachusetts, USA, 2–51, 1995.
Birge, J. R. and Louveaux, F. V.: Introduction to Stochastic Dynamic Programming, Academic Press, Berkeley, California, 2–45, 1997.
Bizon, N.: Real-time optimization strategies of Fuel Cell Hybrid Power Systems based on Load-following control: A new strategy, and a comparative study of topologies and fuel economy obtained, Appl. Energ., 241, 444–460, https://doi.org/10.1016/j.apenergy.2019.03.026, 2019.
Borhan, H., Vahidi, A., Phillips, A. M., Kuang, M. L., Kolmanovsky, I. V., and Di Cairano, S.: MPC-Based Energy Management of a Power-Split Hybrid Electric Vehicle, IEEE T. Contr. Syst. T., 20, 593–603, https://doi.org/10.1109/TCST.2011.2134852, 2012.
Boyd, S. and Vandenberghe, L.: Convex Optimization, IEEE Trans. Automat. Contr., 51, 1859, https://doi.org/10.1109/TAC.2006.884922, 2006.
Cairano, S. D., Bernardini, D., Bemporad, A., and Kolmanovsky, I. V.: Stochastic MPC With Learning for Driver-Predictive Vehicle Control and its Application to HEV Energy Management, IEEE T. Contr. Syst. T., 22, 1018–1031, https://doi.org/10.1109/TCST.2013.2272179, 2014.
Chen, B. and Evangelou, S. A.: Truncated Battery Power Following Strategy for Energy Management Control of Series Hybrid Electric Vehicles, in: Proceedings of European Control Conference (UCC), Napoli, Italy, 25–28 June 2019, https://doi.org/10.23919/ECC.2019.8795784, 2019.
Chen, H., Kessels, J., and Weiland, S.: Online adaptive approach for a game-theoretic strategy for complete vehicle energy management, in: Proceedings of 2015 European Control Conference (ECC), Linz, Austria, 15–17 July 2015, https://doi.org/10.1109/ECC.2015.7330535, 2015.
Chen, L., Liao, Z., Ma, X., and Liu, C.: Hierarchical Control-based Real-time Energy Management Strategy for Hybrid Electric Vehicles, Acta Armamentarii, 42, 1580–1591, https://doi.org/10.3969/j.issn.1000-1093.2021.08.002, 2021.
Chen, Z., Mi, C., Xiong, R., Xu, J., and You, C.: Energy management of a power-split plug-in hybrid electric vehicle based on genetic algorithm and quadratic programming, J. Power Sources, 248, 416–426, https://doi.org/10.1016/j.jpowsour.2013.09.085, 2014.
Chen, Z., Yang, C., and Fang, S.: A Convolutional Neural Network-Based Driving Cycle Prediction Method for Plug-in Hybrid Electric Vehicles With Bus Route, IEEE Access, 8, 3255–3264, https://doi.org/10.1109/ACCESS.2019.2960771, 2020.
Cheng, M. and Chen, B.: Nonlinear Model Predictive Control of a Power-Split Hybrid Electric Vehicle with Consideration of Battery Aging, J. Dyn. Syst.-T. ASME, 141, 081008, https://doi.org/10.1115/1.4042954, 2019.
Delkhosh, M., Foumani, M. S., and Azad, N. L.: A New Framework for Advancement of Power Management Strategies in Hybrid Electric Vehicles, Int. J. Eng. Sci., 33, 468–476, https://doi.org/10.5829/ije.2020.33.03c.11, 2020.
Denis, N., Dubois, M. R., and Desrochers, A.: Fuzzy-based blended control for the energy management of a parallel plug-in hybrid electric vehicle, IET Intell. Transp. Sy., 9, 30–37, https://doi.org/10.1049/iet-its.2014.0075, 2015.
Dextreit, C. and Kolmanovsky, V.: Game Theory Controller for Hybrid Electric Vehicles, IEEE T. Contr. Syst. T., 22, 652–663, https://doi.org/10.1109/TCST.2013.2254597, 2014.
Dougherty, M.: A review of neural networks applied to transport, Transport. Res. C-Emer., 3, 247–260, https://doi.org/10.1016/0968-090X(95)00009-8, 1995.
Du, A., Chen, Y., Zhang, D., and Han, Y.: Multi-Objective Energy Management Strategy Based on PSO Optimization for Power-Split Hybrid Electric Vehicles, Energies, 14, 2438, https://doi.org/10.3390/en14092438, 2021.
Du, Y., Zhao, Y., Wang, Q., Zhang, Y., and Xia, H.: Trip-oriented stochastic optimal energy management strategy for plug-in hybrid electric bus, Energy, 115, 1259–1271, https://doi.org/10.1016/j.energy.2016.09.056, 2016.
Elbert, P., Widmer, M., Gisler, H., and Onder, C.: Stochastic dynamic programming for the energy management of a serial hybrid electric bus, Int. J. Vehicle Des., 69, 88–112, https://doi.org/10.1504/ijvd.2015.073115, 2015.
Freudiger, D., D'Arpino, M., and Canova, M.: Optimal Energy and Thermal Management of Hybrid Battery Packs Using Convex Optimization, in: Proceedings of 2020 American Control Conference, Denver, CO, USA, 1–3 July 2020, https://doi.org/10.23919/ACC45564.2020.9147874, 2020.
Geng, C., Jin, X., and Zhang, X.: Simulation research on a novel control strategy for fuel cell extended-range vehicles, Int. J. Hydrogen Energ., 44, 408–420, https://doi.org/10.1016/j.ijhydene.2018.04.038, 2019.
Gong, Q., Li, Y., and Peng, Z.: Trip-Based Optimal Power Management of Plug-in Hybrid Electric Vehicle, IEEE T. Veh. Technol., 57, 3393–3401, https://doi.org/10.1109/TVT.2008.921622, 2008.
Gu, W., Zhao, D., and Mason, B.: A Review of Intelligent Road Preview Methods for Energy Management of Hybrid Vehicles, IFAC PapersOnLine, 52, 654–660, https://doi.org/10.1016/j.ifacol.2019.09.104, 2019.
Guercioni, G. R., Galvagno, E., Tota, A., and Vigliani, A.: Adaptive Equivalent Consumption Minimization Strategy with Rule-Based Gear Selection for the Energy Management of Hybrid Electric Vehicles Equipped with Dual Clutch Transmissions, IEEE Access, 8, 190017–190038, https://doi.org/10.1109/ACCESS.2020.3032044, 2020.
Guo, J., He, H., Peng, J., and Zhou, N.: A novel MPC-based adaptive energy management strategy in plug-in hybrid electric vehicles, Energy, 175, 378–392, https://doi.org/10.1016/j.energy.2019.03.083, 2019a.
Guo, J., He, H., and Sun, C.: ARIMA-Based Road Gradient and Vehicle Velocity Prediction for Hybrid Electric Vehicle Energy Management, IEEE T. Veh. Technol., 68, https://doi.org/10.1109/TVT.2019.2912893, 2019b.
Guo, L., Gao, B., Gao, Y., and Chen, H.: Optimal Energy Management for HEVs in Eco-Driving Applications Using Bi-Level MPC, IEEE T. Intell. Transp., 18, 2153–2162, https://doi.org/10.1109/TITS.2016.2634019, 2017.
Hadj-Said, S., Colin, G., Ketfi-Cherif, A., and Chamaillard, Y.: Convex Optimization for Energy Management of Parallel Hybrid Electric Vehicles, IFAC PapersOnLine, 49, 271–276, https://doi.org/10.1016/j.ifacol.2016.08.041, 2016.
Hadj-Said, S., Colin, G., Ketfi-Cherif, A., and Chamaillard, Y.: Analytical Solution for Energy Management of Parallel Hybrid Electric Vehicles, IFAC PapersOnLine, 50, 13872–13877, https://doi.org/10.1016/j.ifacol.2017.08.2234, 2017.
Hadj-Said, S., Colin, G., Ketfi-Cherif, A., and Chamaillard, Y.: Energy Management of a Parallel Hybrid Electric Vehicle Equipped with a Voltage Booster, IFAC PapersOnLine, 51, 606–611, https://doi.org/10.1016/j.ifacol.2018.10.145, 2018.
Han, L., Jiao, X., and Zhang, Z.: Recurrent Neural Network-Based Adaptive Energy Management Control Strategy of Plug-In Hybrid Electric Vehicles Considering Battery Aging, Energies, 13, 202, https://doi.org/10.3390/en13010202, 2020.
Han, S., Zhang, F., and Xi, J.: A Real-Time Energy Management Strategy Based on Energy Prediction for Parallel Hybrid Electric Vehicles, IEEE Access, 6, 70313–70323, https://doi.org/10.1109/ACCESS.2018.2880751, 2018.
Han, X., He, H., Wu, J., Peng, J., and Li, Y.: Energy management based on reinforcement learning with double deep Q-learning for a hybrid electric tracked vehicle, Appl. Energ., 254, 113708, https://doi.org/10.1016/j.apenergy.2019.113708, 2019.
Hannan, M. A., Azidin, F. A., and Mohamed, A.: Hybrid electric vehicles and their challenges: A review, Renew. Sust. Energ. Rev., 29, 135–150, https://doi.org/10.1016/j.rser.2013.08.097, 2014.
Hao, J., Yu, Z., Zhao, Z., Shen, P., and Zhan, X.: Optimization of Key Parameters of Energy Management Strategy for Hybrid Electric Vehicle Using DIRECT Algorithm, Energies, 9, 997, https://doi.org/10.3390/en9120997, 2016.
Haußmann, M., Barroso, D., Vidal, C., Bruck, L., and Emadi, A.: A Novel Multi-Mode Adaptive Energy Consumption Minimization Strategy for P1-P2 Hybrid Electric Vehicle Architectures, in: Proceedings of 2019 IEEE Transportation Electrification Conference and Expo (ITEC), Detroit, MI, USA, 19–21 June 2019, https://doi.org/10.1109/ITEC.2019.8790525, 2019.
He, H., Wang, Y., Li, J., Dou, J., Lian, R., and Li, Y.: An Improved Energy Management Strategy for Hybrid Electric Vehicles Integrating Multi-states of Vehicle-Traffic Information, IEEE T. Transp. Electr., 7, 1161–1172, https://doi.org/10.1109/TTE.2021.3054896, 2021.
Hemi, H., Jamel, G., and Ahmed, C.: A real time fuzzy logic power management strategy for a fuel cell vehicle, Energ. Convers. Manage., 80, 63–70, https://doi.org/10.1016/j.enconman.2013.12.040, 2014.
Hu, J., Shao, Y., Sun, Z., Wang, M., Bared, J., and Huang, P.: Integrated optimal eco-driving on rolling terrain for hybrid electric vehicle with vehicle-infrastructure communication, Transport. Res. C-Emer., 68, 228–244, https://doi.org/10.1016/j.trc.2016.04.009, 2016.
Hu, X., Murgovski, N., Johannesson, L., and Egardt, B.: Energy efficiency analysis of a series plug-in hybrid electric bus with different energy management strategies and battery sizes, Appl. Energ., 111, 1001–1009, https://doi.org/10.1016/j.apenergy.2013.06.056, 2013.
Hu, X., Moura, S. J., Murgovski, N., Egardt, B., and Cao, D.: Integrated Optimization of Battery Sizing, Charging, and Power Management in Plug-In Hybrid Electric Vehicles, IEEE T. Contr. Syst. T., 24, 1036–1043, https://doi.org/10.1109/TCST.2015.2476799, 2016.
Hu, X., Liu, T., Qi, X., and Barth, M.: Reinforcement Learning for Hybrid and Plug-In Hybrid Electric Vehicle Energy Management: Recent Advances and Prospects, IEEE Ind. Electron. M., 13, 16–25, https://doi.org/10.1109/MIE.2019.2913015, 2019.
Hu, X., Zou, C., Tang, X., Liu, T., and Hu, L.: Cost-Optimal Energy Management of Hybrid Electric Vehicles Using Fuel Cell/Battery Health-Aware Predictive Control, IEEE T. Power Electr., 35, 382–392, https://doi.org/10.1109/TPEL.2019.2915675, 2020.
Hu, Y., Li, W., Xu, K., Zahid, T., Qin, F., and Li, C.: Energy Management Strategy for a Hybrid Electric Vehicle Based on Deep Reinforcement Learning, Appl. Sci., 8, 187, https://doi.org/10.3390/app8020187, 2018.
Huang, Y., Wang, H., Khajepour, A., He, H., and Ji, J.: Model predictive control power management strategies for HEVs: A review, J. Power Sources, 341, 91–106, https://doi.org/10.1016/j.jpowsour.2016.11.106, 2017.
Husain, I.: Electric and Hybrid Vehicles: Design Fundementals, CRC Press Taylor & Francis Group, London, UK, 2005.
Jager, R.: Fuzzy Logic in Control, PhD thesis, Delft University of Technology, Delft, the Netherlands, 312 pp., http://resolver.tudelft.nl/uuid:418ca337-3518-4a0c-ba87-6117d114cf94 (last access: 8 March 2022), 1995.
Jalil, N., Kheir, N. A., and Salman, M.: A rule-based energy management strategy for a series hybrid vehicle, in: Proceedings of the 1997 American Control Conference, Albuquerque, USA, 6 June 1997, https://doi.org/10.1109/ACC.1997.611889, 1997.
Jeoung, H., Lee, K., and Kim, N.: Methodology for Finding Maximum Performance and Improvement Possibility of Rule-Based Control for Parallel Type-2 Hybrid Electric Vehicles, Energies, 12, 1924, https://doi.org/10.3390/en12101924, 2019.
Jing, P.: Research on Equivalent Fuel Consumption Minimization Strategy of Parallel Hybrid Electric Vehicle, MS thesis, Dalian Maritime University, Dalian, China, 78 pp., 2020.
Jing, P., Wang, X., Cai, M., and Sheng, Y.: Research on an Improved equivalent fuel consumption minimization strategy Based on Ant Colony Algorithm, in: Proceedings of 2019 Chinese Automation Congress (CAC), Hangzhou, China, 22–24 November 2019, https://doi.org/10.1109/CAC48633.2019.8996647, 2019.
Kalia, A. V. and Fabien, B. C.: On Implementing Optimal Energy Management for EREV Using Distance Constrained Adaptive Real-Time Dynamic Programming, Electronics, 9, 228, https://doi.org/10.3390/electronics9020228, 2020.
Khodabakhshian, M., Feng, L., and Wikander, J.: Improving fuel economy and robustness of an improved ECMS method, in: Proceedings of 2013 10th IEEE International Conference on Control and Automation (ICCA), Hangzhou, China, 12–14 June 2013, https://doi.org/10.1109/ICCA.2013.6564946, 2013.
Kim, N., Cha, S., and Peng, H.: Optimal Control of Hybrid Electric Vehicles Based on Pontryagin's Minimum Principle, IEEE T. Contr. Syst. T., 19, 1279–1287, https://doi.org/10.1109/TCST.2010.2061232, 2011.
Kim, N., Jeong, J., and Zheng, C.: Adaptive Energy Management Strategy for Plug-in Hybrid Electric Vehicles with Pontryagin's Minimum Principle Based on Daily Driving Patterns, Int. J. Pr. Eng. Man-GT., 6, 539–548, https://doi.org/10.1007/s40684-019-00046-z, 2019.
Kommuri, N. K., McGordon, A., Allen, A., and Quang, T. D.: Evaluation of a Modified Equivalent Fuel-Consumption Minimization Strategy Considering Engine Start Frequency and Battery Parameters for a Plugin Hybrid Two-Wheeler, Energies, 13, 3122, https://doi.org/10.3390/en13123122, 2020.
Kong, H., Fang, Y., Fan, L., Wang, H., and Hu, J.: A Novel Torque Distribution Strategy Based on Deep Recurrent Neural Network for Parallel Hybrid Electric Vehicle, IEEE Access, 7, 65174–65185, https://doi.org/10.1109/ACCESS.2019.2917545, 2019.
Krithika, V. and Subramani, C.: A comprehensive review on choice of hybrid vehicles and power converters, control strategies for hybrid electric vehicles, Int. J. Energ. Res., 42, 1789–1812, https://doi.org/10.1002/er.3952, 2018.
Larsson, V., Johannesson, L., and Egardt, B.: Analytic Solutions to the Dynamic Programming Subproblem in Hybrid Vehicle Energy Management, IEEE T. Veh. Technol., 64, 1458–1467, https://doi.org/10.1109/TVT.2014.2329864, 2015.
Lee, W., Jeoung, H., Park, D., and Kim, N.: An Adaptive Concept of PMP-Based Control for Saving Operating Costs of Extended-Range Electric Vehicles, IEEE T. Veh. Technol., 68, 11505–11512, https://doi.org/10.1109/TVT.2019.2942383, 2019.
Lei, Z., Qin, D., Hou, L., Peng, J., Liu, Y., and Chen, Z.: An adaptive equivalent consumption minimization strategy for plug-in hybrid electric vehicles based on traffic information, Energy, 190, 116409, https://doi.org/10.1016/j.energy.2019.116409, 2020.
Li, C.: Hybrid Electric Vehicle Energy Management Based on Cruise Speed Optimization, MS degree, Jilin University, Changchun, China, 71 pp., 2016.
Li, G. and Görges, D.: Ecological Adaptive Cruise Control and Energy Management Strategy for Hybrid Electric Vehicles Based on Heuristic Dynamic Programming, IEEE T. Intell. Transp., 20, 3526–3535, https://doi.org/10.1109/TITS.2018.2877389, 2019a.
Li, G. and Görges, D.: Fuel-Efficient Gear Shift and Power Split Strategy for Parallel HEVs Based on Heuristic Dynamic Programming and Neural Networks, IEEE T. Veh. Technol., 68, 9519–9528, https://doi.org/10.1109/TVT.2019.2927751, 2019b.
Li, H., Wei, D., Fu, B., Zou, S., and Zhou, Y.: Energy Management Strategy for A CVT Hybrid Electric Vehicle Based on Dynamic Programming, in: Proceedings of 2019 5th International Conference on Control, Automation and Robotics (ICCAR), Beijing, China, 19–22 April 2019, https://doi.org/10.1109/ICCAR.2019.8813496, 2019.
Li, L., You, S., Yang, C., Yan, B., Song, J., and Chen, Z.: Driving-behavior-aware stochastic model predictive control for plug-in hybrid electric buses, Appl. Energ., 162, 868–879, https://doi.org/10.1016/j.apenergy.2015.10.152, 2016.
Li, P., Jiao, X., and Li, Y.: Adaptive real-time energy management control strategy based on fuzzy inference system for plug-in hybrid electric vehicles, Control Eng. Pract., 107, 104703, https://doi.org/10.1016/j.conengprac.2020.104703, 2021.
Li, S.: Research on Energy Management Strategy of Series Hybrid Vehicle, MS thesis, Beijing Institute of Technology, Beijing, China, 72 pp., 2019.
Li, S., Hu, M., Gong, C., Zhan, S., and Qin, D.: Energy Management Strategy for Hybrid Electric Vehicle Based on Driving Condition Identification Using KGA-Means, Energies, 11, 1531, https://doi.org/10.3390/en11061531, 2018.
Li, T., Rizzoni, G., and Onori, S.: Energy Management Strategy for HEVs Including Battery Life Optimization, IEEE T. Transp. Electr., 1, 211–222, https://doi.org/10.1109/TTE.2015.2471180, 2015.
Li, W.: Research of Hybrid Electric Vehicle Control System and Energy Management Strategy, PhD thesis, Shanghai Jiao Tong University, Shanghai, China, 110 pp., 2008.
Li, X., Wang, Y., Yang, D., and Chen, Z.: Adaptive Energy Management Strategy for Fuel Cell/Battery Hybrid Vehicles using Pontryagin's Minimal Principle, J. Power Sources, 440, 227105, https://doi.org/10.1016/j.jpowsour.2019.227105, 2019.
Li, Y. and Jiao, X.: Real-time energy management for commute HEVs using modified A-ECMS with traffic information recognition, IET Intell. Transp. Sy., 13, 729–737, https://doi.org/10.1049/iet-its.2018.5274, 2019.
Li, Y., Jiao, X., and Jing, Y.: A real-time energy management strategy combining rule-based control and ECMS with optimization equivalent factor for HEVs, in: Proceedings of 2017 Chinese Automation Congress (CAC), Jinan, China, 20–22 October 2017, https://doi.org/10.1109/CAC.2017.8243855, 2017.
Lian, J., Liu, S., Li, L., Liu, X., Zhou, Y., Yang, F., and Yuan, L.: A Mixed Logical Dynamical-Model Predictive Control (MLD-MPC) Energy Management Control Strategy for Plug-in Hybrid Electric Vehicles (PHEVs), Energies, 10, 74, https://doi.org/10.3390/en10010074, 2017.
Lian, R., Peng, J., Wu, Y., Tan, H., and Zhang, H.: Rule-interposing deep reinforcement learning based energy management strategy for power-split hybrid electric vehicle, Energy, 197, 117297, https://doi.org/10.1016/j.energy.2020.117297, 2020.
Liu, G. and Zhang, J.: An energy management of plug-in hybrid electric vehicles based on driver behavior and road information, J. Intell. Fuzzy Syst., 33, 3009–3020, https://doi.org/10.3233/JIFS-169352, 2017.
Liu, H., Li, X., Wang, W., Wang, Y., Han, L., and Wei, W.: Energy management strategy based on GIS information and MPC for a heavy-duty dual-mode power-split HEV, in: Proceedings of 2018 3rd International Conference on Advanced Robotics and Mechatronics (ICARM), Singapore, Singapore, 18–20 July 2018, https://doi.org/10.1109/ICARM.2018.8610835, 2018a.
Liu, H., Wang, C., Zhao, X., and Guo, C.: An Adaptive-Equivalent Consumption Minimum Strategy for an Extended-Range Electric Bus Based on Target Driving Cycle Generation, Energies, 11, 1805, https://doi.org/10.3390/en11071805, 2018b.
Liu, J., Chen, Y., Zhan, J., and Shang, F.: Heuristic Dynamic Programming Based Online Energy Management Strategy for Plug-In Hybrid Electric Vehicles, IEEE T. Veh. Technol., 68, 4479–4493, https://doi.org/10.1109/TVT.2019.2903119, 2019.
Liu, T., Hu, X., Eben, Li S., and Cao, D.: Reinforcement Learning Optimized Look-Ahead Energy Management of a parallel Hybrid Electric Vehicle, IEEE-ASME T. Mech., 22, 1497–1507, https://doi.org/10.1109/TMECH.2017.2707338, 2017.
Liu, T., Yu, H., Guo, H., Qin, Y., and Zou, Y.: Online Energy Management for Multimode Plug-in Hybrid Electric Vehicles, IEEE T. Ind. Inform., 15, 4352–4361, https://doi.org/10.1109/TII.2018.2880897, 2018a.
Liu, T., Wang, B., and Yang, C.: Online Markov Chain-based energy management for a hybrid tracked vehicle with speedy Q-learning, Energy, 160, 544–555, https://doi.org/10.1016/j.energy.2018.07.022, 2018b.
Liu, T., Tan, W., Tang, X., Chen, J., and Cao, D.: Adaptive Energy Management for Real Driving Conditions via Transfer Reinforcement Learning, arXiv [preprint], arXiv:2007.12560, 24 July 2020.
Liu, Y., Huang, Z., Li, J., Ye, M., Zhang, Y., and Chen, Z.: Cooperative optimization of velocity planning and energy management for connected plug-in hybrid electric vehicles, Appl. Math. Model., 95, 715–733, https://doi.org/10.1016/j.apm.2021.02.033, 2021.
Lü, X., Wu, Y., Lian, J., Zhang, Y., Chen, C., Wang, P., and Meng, L.: Energy management of hybrid electric vehicles: A review of energy optimization of fuel cell hybrid power system based on genetic algorithm, Energ. Convers. Manage., 205, 112474, https://doi.org/10.1016/j.enconman.2020.112474, 2020.
Luo, C., Shen, Z., Evangelou, S., Xiong, G., and Wang, F.: The Combination of Two Control Strategies for Series Hybrid Electric Vehicle, IEEE-CAA J. Automatic, 6, 596–608, https://doi.org/10.1109/JAS.2019.1911420, 2019.
Luo, Y., Chen, T., Zhang, S., and Li, K.: Intelligent hybrid electric vehicle ACC with coordinated control of tracking ability, fuel economy, and ride comfort, IEEE T. Intell. Transp., 16, 2303–2308, https://doi.org/10.1109/TITS.2014.2387356, 2015.
Ma, K., Wang, Z., Liu, H., Yu, H., and Wei, C.: Numerical Investigation on Fuzzy Logic Control Energy Management Strategy of Parallel Hybrid Electric Vehicle, Enrg. Proced., 158, 2643–2648, https://doi.org/10.1016/j.egypro.2019.02.016, 2019.
Mahyiddin, S. H., Mohamed, M., Mustaffa, R., Khor, A. C., Sulaiman, M. H., Ahmad, H., and Abdul, R. S.: Fuzzy logic energy management system of series hybrid electric vehicle, in: Proceedings of the 4th IET Clean Energy and Technology Conference (CEAT 2016), Kuala Lumpur, Malaysia, 14–15 November 2016, https://doi.org/10.1049/cp.2016.1267, 2016.
Majed, C., Karaki, S. H., and Jabr, R.: Neural network technique for hybrid electric vehicle optimization, in: Proceedings of 2016 18th Mediterranean Electrotechnical Conference, Lemesos, Cyprus, 18–20 April 2016, https://doi.org/10.1109/MELCON.2016.7495327, 2016.
Martínez, C. M., Hu, X., Cao, D., Efstathios, V., Gao, B., and Matthias, W.: Energy Management in Plug-in Hybrid Electric Vehicles: Recent Progress and a Connected Vehicles Perspective, IEEE T. Veh. Technol., 66, 4534–4549, https://doi.org/10.1109/TVT.2016.2582721, 2017.
Meng, D., Yu, Z., Zhou, M., and Na, R.: Intelligent fuzzy energy management research for a uniaxial parallel hybrid electric vehicle, Comput. Electr. Eng., 58, 447–464, https://doi.org/10.1016/j.compeleceng.2016.03.014, 2017.
Mesbah, A.: Stochastic Model Predictive Control: An Overview and Perspectives for Future Research, IEEE Contr. Syst. Mag., 36, 30–44, https://doi.org/10.1109/MCS.2016.2602087, 2016.
Miller, J. M.: Hybrid electric vehicle propulsion system architectures of the e-CVT type, IEEE T. Power Electr., 21, 756–767, https://doi.org/10.1109/TPEL.2006.872372, 2006.
Miller, J. M.: Propulsion systems for hybrid vehicles, 2nd ed., The Institution of Engineering and Technology, London, UK, 3–107, 2010.
Mohamed, E. H., Ines, B. S., and Lilia, E. A.: Analysis of rule-based parameterized control strategy for a HEV Hybrid Electric Vehicle, in: Proceedings of 2019 19th international conference on Sciences and Techniques of Automatic control & computer engineering, Sousse, Tunisia, 24–26 March 2019, https://doi.org/10.1109/STA.2019.8717250, 2019.
Mohammad, Z., Morteza, A. N., Ali, H., Majid, K., and Harold, R. C.: Using Adaptive Fuzzy Logic for Intelligent Energy Management in Hybrid Vehicles, in: Proceedings of 2020 28th Iranian Conference on Electrical Engineering (ICEE), Tabriz, Iran, August 2020, https://doi.org/10.1109/ICEE50131.2020.9260941, 2020.
Morari, M. and Baric, M.: Recent developments in the control of constrained hybrid systems, Comput. Chem. Eng., 30, 1619–1631, https://doi.org/10.1016/j.compchemeng.2006.05.041, 2006.
Murgovski, N., Johannesson, L. M., and Sjöberg, J.: Engine On/Off Control for Dimensioning Hybrid Electric Powertrains via Convex Optimization, IEEE T. Veh. Technol., 62, 2949–2962, https://doi.org/10.1109/TVT.2013.2251920, 2013.
Musardo, C., Rizzoni, G., and Staccia, B.: A-ECMS: An Adaptive Algorithm for Hybrid Electric Vehicle Energy Management, in: Proceedings of the 44th IEEE Conference on Decision and Control and 2005 European Control Conference, Seville, Spain, 12–15 December 2005, https://doi.org/10.1109/CDC.2005.1582424, 2005.
Nguyen, B. H., German, R., Trovão, J. P. F., and Bouscayrol, A.: Real-time energy management of battery/supercapacitor electric vehicles based on an adaptation of pontryagin's minimum principle, IEEE T. Veh. Technol., 68, 203–212, https://doi.org/10.1109/TVT.2018.2881057, 2018.
Nüesch, T., Cerofolin, A., Mancini, G., Cavina, N., Onder, C., and Guzzella, L.: Equivalent Consumption Minimization Strategy for the Control of Real Driving NOx Emissions of a Diesel Hybrid Electric Vehicle, Energies, 7, 3148–3178, https://doi.org/10.3390/en7053148, 2014a.
Nüesch, T., Elbert, P., Flankl, M., Onder, C., and Guzzella, L.: Convex Optimization for the Energy Management of Hybrid Electric Vehicles Considering Engine Start and Gearshift Costs, Energies, 7, 834–856, https://doi.org/10.3390/en7020834, 2014b.
Onori, S. and Serrao, L.: On Adaptive-ECMS strategies for hybrid electric vehicles, in: Proceedings of International Scientific Conference on Hybrid and Electric Vehicles, 6–7 December 2011, Malmaison, France, 2011.
Onori, S. and Tribioli, L.: Adaptive Pontryagin's Minimum Principle supervisory controller design for the plug-in hybrid GM Chevrolet Volt, Appl. Energ., 147, 224–234, https://doi.org/10.1016/j.apenergy.2015.01.021, 2015.
Opila, D. F., Wang, X., McGee, R., Gillespie, R. B., Cook, J. A., and Grizzle, J. W.: An Energy Management Controller to Optimally Trade Off Fuel Economy and Drivability for Hybrid Vehicles, IEEE T. Contr. Syst. T., 20, 1490–1504, https://doi.org/10.1109/TCST.2011.2168820, 2012.
Opila, D. F., Wang, X., McGee, R., and Grizzle, J. W.: Real-time implementation and hardware testing of a hybrid vehicle energy management controller based on stochastic dynamic programming, J. Dyn. Syst-T. ASME, 135, 021002, https://doi.org/10.1115/1.4007238, 2013.
Padmarajan, B. V., Mcgordon, A., and Jennings, P. A.: Blended Rule-Based Energy Management for PHEV: System Structure and Strategy, IEEE T. Veh. Technol., 65, 8757–8762, https://doi.org/10.1109/TVT.2015.2504510, 2016.
Pam, A., Bouscayrol, A., Fiani, P., Faval, F., and Barraed, P.: Integration of the Road Slope in the Optimization of the Energy Management Strategy of a Parallel HEV, IFAC PapersOnLine, 52, 28–33, https://doi.org/10.1016/j.ifacol.2019.09.005, 2019.
Panday, A. and Bansal, H. O.: A Review of Optimal Energy Management Strategies for Hybrid Electric Vehicle, IEEE T. Veh. Technol., 2014, 160510, https://doi.org/10.1155/2014/160510, 2014.
Panday, A. and Bansal, H. O.: Energy management strategy for hybrid electric vehicles using genetic algorithm, J. Renew. Sustain. Ener., 8, 741–646, https://doi.org/10.1063/1.4938552, 2016.
Park, S. and Ahn, C.: Power Management Controller for a Hybrid Electric Vehicle with Predicted Future Acceleration. IEEE T. Veh. Technol., 68, 10477–10488, https://doi.org/10.1109/TVT.2019.2939344, 2019.
Peng, H. and Xie, J.: Energy Management Strategy for Plug-In Hybrid Electric Vehicles Based on Genetic- Fuzzy Control strategy, in: Proceedings of 2017 International Conference on Computer Technology, Electronics and Communication (ICCTEC), Dalian, China, 19–21 December 2017, https://doi.org/10.1109/ICCTEC.2017.00231, 2017.
Peng, J.: Research on Collaborative Optimization Energy Management Strategy for Connected and Automated Plug-In Hybrid Electric Vehicle, MS thesis, Chongqing University, Chongqing, China, 109 pp., 2019.
Peng, J., Fan, H., He, H., and Pan, D.: A Rule-Based Energy Management Strategy for a Plug-in Hybrid School Bus Based on a Controller Area Network Bus, Energies, 8, 5122–5142, https://doi.org/10.3390/en8065122, 2015.
Qi, X., Wu, G., Peng, H., Kanok, B., and Matthew, J. B.: Integrated Connected Eco-Driving System for PHEVs with Co-Optimization of Vehicle Dynamics and Powertrain Operations, IEEE T. Int. Veh., 2, 2–13, https://doi.org/10.1109/TIV.2017.2708599, 2017.
Qi, Y., Wang, W., and Xiang, C.: Neural network and efficiency-based control for dual-mode hybrid electric vehicles, in: Proceedings of 2015 34th Chinese Control Conference (CCC), Hangzhou, China, 28–30 July 2015, https://doi.org/10.1109/ChiCC.2015.7260929, 2015.
Qi, Y., Wang, W., Xiang, C., and Zhao, Y.: Economic MPC-based transient control for a dual-mode power-split HEV, Sci. China Ser. E, 60, 1917–1934, https://doi.org/10.1007/s11431-017-9128-4, 2017.
Qian, L., Qiu, L., Lin, R., and Xin, F.: Hierarchical energy management and optimization of hybrid electric vehicles based on V2X, Transactions of the CSAE, 32, 74–81, https://doi.org/10.11975/j.issn.1002-6819.2016.19.010, 2016.
Qian, L., Qiu, L., Si, Y., and Wang, J.: Hierarchical Energy Management of Hybrid Electric Vehicles Under the Environment of Intelligent and Connected Vehicles, Automot. Eng., 39, 621–629, https://doi.org/10.19562/j.chinasae.qcgc.2017.06.003, 2017.
Qian, L., Jing, H., and Qiu, L.: Energy Management of a 4WD HEV Based on SMPC, China Mechanical Engineering, 29, 1342–1348, https://doi.org/10.3969/j.issn.1004-132X.2018.11.014, 2018.
Qiao, Y., Song, Y., and Huang, K.: A Novel Control Algorithm Design for Hybrid Electric Vehicles Considering Energy Consumption and Emission Performance, Energies, 12, 2698, https://doi.org/10.3390/en12142698, 2019.
Qin, F., Xu, G., Hu, Y., Xu, K., and Li, W.: Stochastic Optimal Control of Parallel Hybrid Electric Vehicles, Energies, 10, 214, https://doi.org/10.3390/en10020214, 2017.
Rios-Torres, J., Liu, J., and Khattak, A.: Fuel consumption for various driving styles in conventional and hybrid electric vehicles: Integrating driving cycle predictions with fuel consumption optimization, Int. J. Sustain. Transp., 13, 123–137, https://doi.org/10.1080/15568318.2018.1445321, 2018.
Sabri, M. F. M., Danapalasingam, K. A., and Rahmat, M. F.: A review on hybrid electric vehicles architecture and energy management strategies, Renew. Sust. Energ. Rev., 53, 1433–1442, https://doi.org/10.1016/j.rser.2015.09.036, 2016.
Sabri, M. F. M., Danapalasingam, K. A., and Rahmat, M. F.: Improved Fuel Economy of Through-the-Road Hybrid Electric Vehicle with Fuzzy Logic-Based Energy Management Strategy, Int. J. Fuzzy Syst., 20, 2677–2692, https://doi.org/10.1007/s40815-018-0521-4, 2018.
Sanchez, M. and Delpra, S.: Hybrid Vehicle Energy Management: Avoiding the Explicit Hamiltonian Minimization, in: Proceedings of 2018 IEEE Vehicle Power & Propulsion Conference (VPPC), Chicago, IL, USA, 27–30 August 2018, https://doi.org/10.1109/VPPC.2018.8604992, 2018.
Shen, Y., Cui, P., Wang, X., Han, X., and Wang, Y.: Variable structure battery-based fuel cell hybrid power system and its incremental fuzzy logic energy management strategy, Int. J. Hydrogen Energ., 45, 12130–12142, https://doi.org/10.1016/j.ijhydene.2020.02.083, 2020.
Shi, D., Wang, S., Cai, Y., and Chen, L.: Stochastic Predictive Energy Management of Power Split Hybrid Electric Bus for Real-World Driving Cycles, IEEE Access, 6, 61700–61713, https://doi.org/10.1109/ACCESS.2018.2876147, 2018.
Singh, K. V., Bansal, H. O., and Singh, D.: A comprehensive review on hybrid electric vehicles: architectures and components, J. Mod. Transport, 27, 77–107, https://doi.org/10.1007/s40534-019-0184-3, 2019.
Singh, K. V., Bansal, H. O., and Singh, D.: Feed-forword modeling and real-time implementation of an intelligent fuzzy logic-based energy management strategy in a series-parallel hybrid electric vehicle to improve fuel economy, Electr. Eng., 102, 967–987, https://doi.org/10.1007/s00202-019-00914-6, 2020.
Singh, K. V., Bansal, H. O., and Singh, D.: Fuzzy logic and Elman neural network tuned energy management strategies for a power-split HEVs, Energy, 225, 120152, https://doi.org/10.1016/j.energy.2021.120152, 2021.
Sölek, H., Müderrisoglu, K., Armutlu, C., and Yilmaz, M.: Development of Fuzzy Logic Based Energy Management Control Algorithm for a Plug-in HEV with Fixed Routed, in: Proceedings of 2019 International Conference on Optimization of Electrical and Electronic Equipment (OPTIM), Istanbul, Turkey, 27–29 August 2019, https://doi.org/10.1109/ACEMP-OPTIM44294.2019.9007192, 2019.
Song, C., Zhou, F., Xiao, F., Chang, C., and Shao, Y.: Parameter Matching of On-board Hybrid Energy Storage System Based on Convex Optimization Method, J. Mech. Eng., 16, 44–51, https://doi.org/10.3901/JME.2017.16.044, 2017.
Sulaiman, N., Hannan, M. A., Mohamed, A., Majlan, E. H., and Daud, W. R.: A review on energy management system for fuel cell hybrid electric vehicle: Issues and challenges, Renew. Sust. Energ. Rev., 52, 802–814, https://doi.org/10.1016/j.rser.2015.07.132, 2015.
Sun, C., Hu, X., Moura, S. J., and Sun, F.: Velocity Predictors for Predictive Energy Management in Hybrid Electric Vehicles, IEEE T. Contr. Syst. T., 23, 1197–1204, https://doi.org/10.1109/TCST.2014.2359176, 2015a.
Sun, C., Moura, S. J., Hu, X., Hedrick, J. K., and Sun, F.: Dynamic Traffic Feedback Data Enabled Energy Management in Plug-in Hybrid Electric Vehicles, IEEE T. Contr. Syst. T., 23, 1075–1086, https://doi.org/10.1109/TCST.2014.2361294, 2015b.
Sun, C., Sun, F., and He, H.: Investigating adaptive-ECMS with velocity forecast ability for hybrid electric vehicles, Appl. Energ., 185, 1644–1653, https://doi.org/10.1016/j.apenergy.2016.02.026, 2016.
Sun, H., Fu, Z., Tao, F., Zhu, L., and Si, P.: Data-driven reinforcement-learning-based hierarchical energy management strategy for fuel cell/battery/ultracapacitor hybrid electric vehicles, J. Power Sources, 455, 227964, https://doi.org/10.1016/j.jpowsour.2020.227964, 2020.
Sutton, R. S. and Barto, A. G.: Reinforcement Learning: An Introduction, IEEE T. Neural Network, 9, 1052, https://doi.org/10.1109/TNN.1998.712192, 1998.
Tian, H.: Self-learning Online Energy Management Strategy for Plug-in Hybrid Electric Bus, Doctor's degree, Tsinghua University, Beijing, 2018b.
Tian, H., Wang, X., Lu, Z., Huang, Y., and Tian, G.: Adaptive Fuzzy Logic Energy Management Strategy Based on Reasonable SOC Reference Curve for Online Control of Plug-in Hybrid Electric City Bus, IEEE T. Intell. Transp., 19, 1607–1617, 2018a.
Tian, X., He, R., Sun, X., Cai, Y., and Xu, Y.: An ANFIS-Based ECMS for Energy Optimization of Parallel Hybrid Electric Bus, IEEE T. Veh. Technol., 69, 1473–1483, https://doi.org/10.1109/TVT.2019.2960593, 2020.
Vafaeipour, M., Baghdadi, M. E., Mierlo, J. V., Hegazy, O., Verbelen, F., and Sergeant, P.: An ECMS-based Approach for Energy Management of a HEV Equipped with an Electrical Variable Transmission, in: Proceedings of 2019 Fourteenth International Conference on Ecological Vehicles and Renewable Energies (EVER), Monte-Carlo, Monaco, 8–10 May 2019, https://doi.org/10.1109/EVER.2019.8813647, 2019.
Wang, C.: Research on Energy Management Strategy of Hybrid Electric Bus Based on Genetic Algorithm, MS thesis, Shandong University, Jinan, China, 62 pp., 2020.
Wang, Q., You, S., Li, L., and Yang, C.: Survey on energy management strategy for plug-in hybrid electric vehicles, J. Mech. Eng., 53, 1–19, https://doi.org/10.3901/JME.2017.16.001, 2017a.
Wang, Q., Du, S., Li, L., You, S., and Yang, C.: Real-time Energy Management Strategy for Plug-in Hybrid Electric Bus on Particle Swarm Optimization Algorithm, J. Mech. Eng., 53, 77–84, https://doi.org/10.3901/JME.2017.04.077, 2017b.
Wang, S. and Qin, D.: Online Control Strategy for Plug-In Hybrid Electric Vehicles Based on an Improved Global Optimization Algorithm, Appl. Sci., 10, 8352, https://doi.org/10.3390/app10238352, 2020.
Wang, S., Li, J., Shi, D., Sun, X., and Yao, Y.: Energy Management Strategy of Dual Planetary Hybrid Electric Vehicle Based on Optimal Transmission Efficiency, J. Theor. App. Mech.-Pol., 57, 383–396, https://doi.org/10.15632/jtam-pl/104591, 2019a.
Wang, S., Huang, X., López, J. M., Xu, X., and Dong, P.: Fuzzy Adaptive-Equivalent Consumption Minimization Strategy for a Parallel Hybrid Electric Vehicle, IEEE Access, 7, 133290–133303, https://doi.org/10.1109/ACCESS.2019.2941399, 2019b.
Wang, Y.: Research on Intelligent Energy Management Strategy for Hybrid Electric Bus Based on Vehicular Network Information, Doctor's degree, Jilin University, Changchun, China, 2020.
Wang, Y., Sun, Z., and Chen, Z.: Development of energy management system based on a rule-based power distribution strategy for hybrid power sources, Energy, 175, 1055–1066, https://doi.org/10.1016/j.energy.2019.03.155, 2019.
Wang, Y., He, H., Peng, J., and Tan, H.: Deep Reinforcement Learning for Plug-in Hybrid Electric Vehicle Energy Management, in: Proceedings of China Society of Automotive Engineering Annual Meeting, Shanghai, China, 27 October 2020, https://kns.cnki.net/kcms/detail/detail.aspx?dbcode=CPFD&dbname=CPFDLAST2020&filename=QCGC202010002093&uniplatform=NZKPT&v=YOfR1kv0lVlBn7DryD97ODDqO7f0LhrAgxiu7r_LuhwbZ8F6nsbKCJcFcWerxH23GPI1qCKQrkI%3d (last access: 8 March 2022), 2020 (in Chinese).
Wang, X., He, H., Sun, F., and Zhang, J.: Application Study on the Dynamic Programming Algorithm for Energy Management of Plug-in Hybrid Electric Vehicles, Energies, 8, 3225–3244, https://doi.org/10.3390/en8043225, 2015.
Wei, Z. and Xu, Z.: D Halim Study of HEV Power Management Control Strategy Based on Driving Pattern Recognition, Enrg. Proced., 88, 847–853, https://doi.org/10.1016/j.egypro.2016.06.062, 2016.
Wu, J., Zhang, C., and Cui, N.: Real-time energy management of parallel hybrid electric vehicle based on BP neural network, electric machines and control, 12, 610–614, https://doi.org/10.3969/j.issn.1007-449X.2008.05.024, 2008 (in Chinese).
Wu, Y., Zhang, Y., Li, G., Shen, J., Chen, Z., and Liu, Y.: A predictive energy management strategy for multi-mode plug-in hybrid electric vehicles based on multi neural networks, Energy, 208, 118366, https://doi.org/10.1016/j.energy.2020.118366, 2020.
Wu, Z.: Energy Management Strategy for Hybrid Electric Vehicle Based on Neural Network and PMP, Master's degree, Southeast University, Nanjing, China, 2018.
Xia, C. and Zhang, C.: Power Management Strategy of Hybrid Electric Vehicles Based on Quadratic Performance Index, Energies, 8, 12458–12473, https://doi.org/10.3390/en81112325, 2015.
Xiang, C., Han, L., Liu, H., and Li, H.: An Analysis on the Characteristics of Power-split and Coupling Mechanism in Series/Parallel HEV, Automot. Eng., 32, 183–187, https://doi.org/10.19562/j.chinasae.qcgc.2010.03.001, 2010.
Xiang, C., Ding, F., Wang, W., and He, W.: Energy management of a dual-mode power-split hybrid electric vehicle based on velocity prediction and nonlinear model predictive control, Appl. Energ., 189, 640–653, https://doi.org/10.1016/j.apenergy.2016.12.056, 2017a.
Xiang, C., Ding, F., Wang, W., He, W., and Qi, Y.: MPC-based energy management with adaptive Markov-chain prediction for a dual-mode hybrid electric vehicle, Sci. China Ser. E, 60, 93–104, https://doi.org/10.1007/s11431-016-0640-2, 2017b.
Xiao, R., Liu, B., Shen, J., Guo, N., Yan, W., and Chen, Z.: Comparisons of Energy Management Methods for a Parallel Plug-In Hybrid Electric Vehicle between the Convex Optimization and Dynamic Programming, Appl. Sci., 8, 218, https://doi.org/10.3390/app8020218, 2018.
Xie, S., He, H., and Peng, J.: An energy management strategy based on stochastic model predictive control for plug-in hybrid electric buses, Appl. Energ., 196, 279–288, https://doi.org/10.1016/j.apenergy.2016.12.112, 2017.
Xie, S., Hu, X., Xin, Z., and Brighton, J.: Pontryagin's Minimum Principle based model predictive control of energy management for a plug-in hybrid electric bus, Appl. Energ., 236, 893–905, https://doi.org/10.1016/j.apenergy.2018.12.032, 2019.
Xiong, R., Cao, J., and Yu, Q.: Reinforcement learning-based real-time power management for hybrid energy storage system in the plug-in hybrid electric vehicle, Appl. Energ., 211, 538–548, https://doi.org/10.1016/j.apenergy.2017.11.072, 2018.
Xu, B., Malmir, F., Rathod, D., and Filipi, Z.: Real-Time Reinforcement Learning Optimized Energy Management for a 48V Mild Hybrid Electric Vehicle, SAE Technical Paper, New York, USA, https://doi.org/10.4271/2019-01-1208, 2019.
Xu, B., Rathoda, D., Zhang, D., Yebia, A., Zhang, X., Li, X., and Filipi, Z.: Parametric study on reinforcement learning optimized energy management strategy for a hybrid electric vehicle, Appl. Energ., 259, 114200, https://doi.org/10.1016/j.apenergy.2019.114200, 2020.
Xu, J., Alsabbagh, A., Yan, D., and Ma, C.: Game-theoretic energy management with velocity prediction in hybrid electric vehicle, in: Proceedings of 28th International Symposium on Industrial Electronics (ISIE), Vancouver, BC, Canada, 12–14 June 2019, https://doi.org/10.1109/ISIE.2019.8781119, 2019.
Xu, J., Alsabbagh, A., and Ma, C.: Prediction-based Game-theoretic Strategy for Energy Management of Hybrid Electric Vehicles, IEEE Journal of Emerging and Selected Topics in Industrial Electronics, 3, 79–89, https://doi.org/10.1109/JESTIE.2021.3087962, 2021.
Xu, Q., Luo, X., Jiang, X., and Zhao, M.: Research on Double Fuzzy Control Strategy for Parallel Hybrid Electric Vehicle Based on GA and DP Optimization, IET Electr. Syst. Transp., 8, 144–151, https://doi.org/10.1049/iet-est.2017.0067, 2018a.
Xu, Q., Mao, Y., Zhao, M., and Cui, S.: A Hybrid Electric Vehicle Dynamic Optimization Energy Management Strategy Based on a Compound-Structured Permanent-Magnet Motor, Energies, 11, 2212, https://doi.org/10.3390/en11092212, 2018b.
Xue, Q., Zhang, X., Teng, T., Zhang, J., Feng, Z., and lv, Q.: A Comprehensive Review on Classification, Energy Management Strategy, and Control Algorithm for Hybrid Electric Vehicles, Energies, 13, 5355, https://doi.org/10.3390/en13205355, 2020.
Yan, F., Wang, J., and Huang, K.: Hybrid Electric Vehicle Model Predictive Control Torque-Split Strategy Incorporating Engine Transient Characteristics, IEEE T. Veh. Technol., 61, 2458–2467, https://doi.org/10.1109/TVT.2012.2197767, 2012.
Yang, C., Li, L., You, S., Yan, B., and Du, X.: Multimode Energy Management for Plug-In Hybrid Electric Buses Based on Driving Cycles Prediction, IEEE T. Intell. Transp., 17, 2811–2821, https://doi.org/10.1109/TITS.2016.2527244, 2016.
Yang, C., Li, L., You, S., Yan, B., and Du, X.: Cloud computing-based energy optimization control framework for plug-in hybrid electric bus, Energy, 125, 11–26, https://doi.org/10.1016/j.energy.2017.02.102, 2017.
Yang, F., Wang, S., Li, J., Liu, Z., and Sun, Q.: An overview of internet of vehicles, China Commun., 11, 1–15, 2014.
Yang, Z., Chen, H., Dong, S., Liu, Q., and Xu, F.: Energy Management Strategy of Hybrid Electric Vehicle with Consideration of Road Gradient, in: 2020 Chinese Control and Decision Conference (CCDC), Hefei, China, 22–24 August 2020, https://doi.org/10.1109/CCDC49329.2020.9163950, 2020.
Yin, A. and Tian, X.: A New HEV Energy Management Based on Game Theory, Auto Sci.-Tech., 4, 49–52, https://doi.org/10.3969/j.issn.1005-2550.2010.04.013, 2010.
Yin, H., Zhao, C., Li, M., Ma, C., and Chow, M.: A Game Theory Approach to Energy Management of an Engine-Generator/Battery/Ultracapacitor Hybrid Energy System, IEEE T. Ind. Electron., 63, 4266–4277, https://doi.org/10.1109/TIE.2016.2539245, 2016.
Yin, H., Zhao, C., and Ma, C.: Decentralized Real-Time Energy Management for A Reconfigurable Multiple-Source Energy System, IEEE T. Ind. Inform., 14, 4128–4137, https://doi.org/10.1109/TII.2018.2827466, 2018.
Yu, X., Cao, S., Li, J., Gao, Y., Yang, S., Zhong, X., and Sun, P.: Present study situation and developing trend of control strategies for hybrid electric vehicle, J. Mech. Eng., 42, 10–16, 2006.
Yuan, Z., Teng, L., Sun, F., and Peng, H.: Comparative Study of Dynamic Programming and Pontryagin's Minimum Principle on Energy Management for a Parallel Hybrid Electric Vehicle, Energies, 6, 2305–2318, https://doi.org/10.3390/en6042305, 2013.
Zeng, X. and Wang, J.: A Parallel Hybrid Electric Vehicle Energy Management Strategy Using Stochastic Model Predictive Control with Road Grade Preview, IEEE T. Contr. Syst. T., 2416–2423, https://doi.org/10.1109/TCST.2015.2409235, 2015.
Zhan, S., Qin, D., and Zeng, Y.: Energy Management Strategy of HEV Based on Driving Cycle Recognition Using Genetic Optimized K-means Clustering Algorithm, China J. Highw. Transp., 29, 130–137, https://doi.org/10.19721/j.cnki.1001-7372.2016.04.016, 2016.
Zhang, B., Xu, F., and Shen, T.: A Real-Time Energy Management Strategy for Parallel HEVs with MPC, in: Proceedings of 2019 IEEE Vehicle Power and Propulsion Conference (VPPC), Hanoi, Vietnam, 14–17 October 2019, https://doi.org/10.1109/VPPC46532.2019.8952384, 2019.
Zhang, B., Guo, S., Zhang, X., Xue, Q., and Teng, L.: Adaptive Smoothing Power Following Control Strategy Based on an Optimal Efficiency Map for a Hybrid Electric Tracked Vehicle, Energies, 13, 1893, https://doi.org/10.3390/en13081893, 2020.
Zhang, F., Liu, H., Hu, Y., and Xi, J.: A Supervisory Control Algorithm of Hybrid Electric Vehicle Based on Adaptive Equivalent Consumption Minimization Strategy with Fuzzy PI, Energies, 9, 919, https://doi.org/10.3390/en9110919, 2016.
Zhang, F., Xi, J., and Reza, L.: Real-Time Energy Management Strategy Based on Velocity Forecasts Using V2V and V2I Communications, IEEE T. Intell. Transp., 18,416-430, https://doi.org/10.1109/TITS.2016.2580318, 2017.
Zhang, F., Hu, X., Xu, K., Tang, X., and Cui, Y.: Current Status and Prospects for Model Predictive Energy Management in Hybrid Electric Vehicles, J. Mech. Eng., 55, 86–108, https://doi.org/10.3901/JME.2019.10.086, 2019.
Zhang, F., Wang, L., Coskun, S., Pang, H., Cui, Y., and Xi, J.: Energy Management Strategies for Hybrid Electric Vehicles: Review, Classification, Comparison, and Outlook, Energies, 13, 3352, https://doi.org/10.3390/en13133352, 2020.
Zhang, J. and Shen, T.: Real-Time Fuel Economy Optimization With Nonlinear MPC for PHEVs, IEEE T. Contr. Syst. T., 24, 2167–2175, https://doi.org/10.1109/TCST.2016.2517130, 2016.
Zhang, P., Wu, X., Du, C., Xu, H., and Wang, H.: Adaptive Equivalent Consumption Minimization Strategy for Hybrid Heavy-Duty Truck Based on Driving Condition Recognition and Parameter Optimization, Energies, 13, 5407, https://doi.org/10.3390/en13205407, 2020.
Zhang, Q. and Fu, X.: A Neural Network Fuzzy Energy Management Strategy for Hybrid Electric Vehicles Based on Driving Cycle Recognition, Appl. Sci., 10, 696, https://doi.org/10.3390/app10020696, 2020.
Zhang, R., Tao, J., and Zhou, H.: Fuzzy Optimal Energy Management for Fuel Cell and Supercapacitor Systems Using Neural Network Based Driving Pattern Recognition, IEEE T. Fuzzy Syst., 27, 45–57, https://doi.org/10.1109/TFUZZ.2018.2856086, 2019.
Zhang, S. and Xiong, R.: Adaptive energy management of a plug-in hybrid electric vehicle based on driving pattern recognition and dynamic programming, Appl. Energ., 155, 68–78, https://doi.org/10.1016/j.apenergy.2015.06.003, 2015.
Zhang, X., Liu, Y., Zhang, J., Dai, W., and Liu, Z.: A Fuzzy Neural Network Energy Management Strategy for Parallel Hybrid Electric Vehicle, in: Proceedings of the 9th International Conference on Modelling, Identification and Control (ICMIC 2017), Kunming, China, 10–12 July 2017, https://doi.org/10.1109/ICMIC.2017.8321666, 2017.
Zhang, Y., Liu, H., and Guo, Q.: Varying-Domain Optimal Management Strategy for Parallel Hybrid Electric Vehicles, IEEE T. Veh. Technol., 63, 603–616, https://doi.org/10.1109/TVT.2013.2276432, 2014.
Zhao, J. and Antonio, S.: Design and Control Co-Optimization for Powertrain: Development of Dedicated Optimal Energy Management Strategy, IFAC PapersOnLine, 49, 277–284, https://doi.org/10.1016/j.ifacol.2016.08.042, 2016.
Zhao, X. and Guo, G.: Survey on energy management strategies for hybrid electric vehicles, Acta Automatica Sinica, 3, 321–334, https://doi.org/10.16383/j.aas.2016.c150477, 2016.
Zheng, C. and Mi, C.: An adaptive online energy management controller for power-split HEV based on Dynamic Programming and fuzzy logic, in: Proceedings of 2009 IEEE Vehicle Power and Propulsion Conference, Dearborn, MI, USA, 7–10 September 2009, https://doi.org/10.1109/VPPC.2009.5289831, 2009.
Zhou, F., Xiao, F., Chang, C., Shao, Y., and Song, C.: Adaptive Model Predictive Control-Based Energy Management for Semi-Active Hybrid Energy Storage Systems on Electric Vehicles, Energies, 10, 1063, https://doi.org/10.3390/en10071063, 2017.
Zhou, H., Xu, Z., Liu, L., Liu, D., and Zhang, L.: A Rule-Based Energy Management Strategy Based on Dynamic Programming for Hydraulic Hybrid Vehicles, Math. Probl. Eng., 14, 1–10, https://doi.org/10.1155/2018/9492026, 2018.
Zhou, W., Liu, H., Xu, B., and Zhang, L.: Predictive Equivalent Consumption Minimization Strategy for Power Split Hybrid Electric Mining Truck, J. Mech. Eng., 57, 200–209, https://doi.org/10.3901/JME.2021.02.200, 2021.
Zou, Y., Liu, T., Liu, D., and Sun, F.: Reinforcement learning-based real-time energy management for a hybrid tracked vehicle, Appl. Energ., 171, 372–382, https://doi.org/10.1016/j.apenergy.2016.03.082, 2016.
Zuo, Y., Xiang, C., and Yan, Q.: Control Strategy of Parallel-serial Hybrid Electrical Vehicle Based on the Power Track Method, T. Chin. Soc. Agric. Mach., 41, 23–29, https://doi.org/10.1109/CLEOE-EQEC.2009.5194697, 2009.
- Abstract
- Introduction
- Research on energy management issues
- The rule-based energy management strategy
- The optimization-based energy management strategy
- The EMS based on intelligent transportation systems
- Conclusion and prospects
- Appendix A: Abbreviation
- Data availability
- Author contributions
- Competing interests
- Disclaimer
- Review statement
- References
- Abstract
- Introduction
- Research on energy management issues
- The rule-based energy management strategy
- The optimization-based energy management strategy
- The EMS based on intelligent transportation systems
- Conclusion and prospects
- Appendix A: Abbreviation
- Data availability
- Author contributions
- Competing interests
- Disclaimer
- Review statement
- References