the Creative Commons Attribution 4.0 License.
the Creative Commons Attribution 4.0 License.
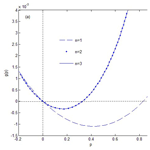
Stochastic stability and the moment Lyapunov exponent for a gyro-pendulum system driven by a bounded noise
Shenghong Li
Junting Lv
The stochastic stability of a gyro-pendulum system parametrically excited by a real noise is investigated by the moment Lyapunov exponent in the paper. Using the spherical polar and non-singular linear stochastic transformations and combining these with Khasminskii's method, the diffusion process and the eigenvalue problem of the moment Lyapunov exponent are obtained. Then, applying the perturbation method and Fourier cosine series expansion, we derive an infinite-order matrix whose leading eigenvalue is the second-order expansion g2(p) of the moment Lyapunov exponent. Thus, an infinite sequence for g2(p) is constructed, and its convergence is numerically verified. Finally, the influences of the system and noise parameters on stochastic stability are given such that the stochastic stability is strengthened with the increased drift coefficient and the diffusion coefficient has the opposite effect; among the system parameters, only the increase in k and A0 strengthens moment stability.
- Article
(1478 KB) - Full-text XML
- BibTeX
- EndNote
There are many definitions of stochastic stability, among which the pth moment stability has attracted a lot of attention. The stability is usually described by the moment Lyapunov exponent, which was first presented in 1984 (Arnold, 1984). Then the moment Lyapunov exponent of the linear systems driven by the real and white noises was given, and the stochastic moment stability of linear system was completely resolved (Arnold et al., 1986a).
However, it is extremely difficult to obtain the analytic expression of the moment Lyapunov exponent for an actual dynamical system according to Arnold's results due to the complexity of the noise and system. So far, almost all the results about moment Lyapunov exponents were published through the approximate analytical methods. The asymptotic expansions of the moment Lyapunov exponents on a weak noise and a small value of p were first applied to analyse the stability of a two-dimensional stochastic system (Arnold et al., 1997). In a similar manner, Namachchivaya et al. (1996) studied the moment Lyapunov exponent for a system with two coupled oscillators excited by a real noise. For a linear conservative system with a white noise, Khasminskii and Moshchuk (1998) proved that both the moment Lyapunov exponent with the finite p and the stability index can only be regarded as the asymptotic expansions of small noise intensity. Referring to the results in a previous paper, for the same system and random excitation as Arnold et al. (1997), the asymptotic expansion of the finite pth moment Lyapunov exponent was also presented (Namachchivaya, 2001). For several two-dimensional systems with the real or bounded noise excitations, Xie (2001a, b, 2003) researched the weak noise expansions of the finite pth moment Lyapunov exponent, the maximal Lyapunov exponent, and the stability index through a similar procedure. The stability properties of a Van der Pol–Duffing oscillator excited by a real noise were investigated (Liu and Liew, 2005). Due to the complexity of approximate analytical methods, Higham et al. (2007) gave the numerical simulation of the moment Lyapunov exponent in stochastic differential equations. Then, the moment Lyapunov exponent and stochastic stability of a double-beam system under the compressive axial loading and moving narrow bands were discussed (Kozic et al., 2010). S. H. Li and X. B. Liu (2012, 2013) studied the moment Lyapunov exponent for a three-dimensional stochastic system based on the perturbation method. Hu et al. (2012, 2017) and X. Li and X. B. Liu (2013, 2014) obtained the moment Lyapunov exponent for a binary airfoil system under coloured noise excitation, which indicates stochastic dynamical theory has extended to the aviation field.
Gyro-pendulums are usually used to stabilise the firing of guns on warships or tanks or to navigate cars and aircraft, and the stability of the gyro-pendulum is a classical topic in dynamics and control, which made some researchers interested in it. The mean square and almost sure stability of a gyro-pendulum under random vertical support and white-noise excitation were researched through the stochastic averaging method (Namachchivaya, 1987; Asokanthan and Ariaratnam, 2000). Recently, the stochastic stability of a planner gyro-pendulum system excited by white noises has been presented (Li and Xu, 2019). However, as we know, the ideal white noise has infinite bandwidth and is difficult to achieve in practice. Therefore, we choose a bound noise to discuss the stochastic stability of a gyro-pendulum system parametrically driven by the bounded noise in this paper. In Sect. 2, the mathematical model is given. Applying a perturbation method, the eigenvalue problem of the moment Lyapunov exponent is obtained in Sect. 3 and solved via a Fourier cosine series expansion in Sect. 4. The numerical results are given in Sect. 5. In Sect. 6, the conclusions are presented.
A typical gyro-pendulum system in the vertical configuration shown in Fig. 1 is considered, and its motion equations with a stochastic excitation f(t) are written as (Namachchivaya, 1987)
where θ1 and θ2 are the motion of the outer gimbal about an inertial co-ordinate system OXYZ and the motion of the inner frame with respect to the y axis of the outer one, respectively. , , A, and C are the inertial moment of the gyro about the rotation axis and any axis perpendicular to Oz. A′ and B′ are the inertial moments of the inner gimbal about the rotor principal axes Ox and Oy, respectively, and A′′ represents the inertial moment of the outer frame about the axis OX. k=mgl denotes the pendulous stiffness, l is the pendulosity of the gyroscope, and n is the spin speed. k1 is the stiffness of the excitation f(t).
For the noise excitation, we introduce a bound noise cos [ξ(t)] because of its rather universal sense in engineering (Li and Wu, 2015; Li and Liu, 2012). Meanwhile, considering the system, damping the system Eq. (1) is rewritten as
where is a very small number. , , a11=d2/B0, a12=Cn/B0, , a22=d1/A0, d1, and d2 are damping coefficients. ; . The symbol “∘” represents that Eq. (2) is a Stratonovich stochastic differential equation, μ is drift coefficient, σ is diffusion coefficient, and both are any real constants; W(t) is a unit Wiener process.
Letting θ1=x1, , θ2=x3, and , Eq. (2) is changed into a vector-matrix equation:
where
Through applying the spherical polar transformation
and substituting them into Eq. (3), the equations for norm process P and phase processes θ, ϕ1, and ϕ2 can be obtained according to Itô's lemma:
where
For the norm process P, a non-singular linear stochastic transformation is introduced, i.e.
where the function is a scalar function of the phase processes . Thus, Itô's stochastic equation for the new norm process S is derived by Itô's lemma:
Since is reversible and bounded, both P and S have the same stability. Therefore, a selection is made such that the drift term of Eq. (6) is independent of the phase processes θ, φ1, φ2 and the noise process ξ; i.e.
Comparing Eqs. (6) and (7), a result yields that is described by the following equation:
The above equation can be written as
where
and its corresponding adjoint operator is
It can be seen from Eqs. (8)–(10) that an eigenvalue problem with the second-order differential operator is defined, where an eigenvalue is just the pth moment Lyapunov exponent g(p) of the system Eq. (4) and is its corresponding eigenfunction.
Furthermore, according to the conclusions presented (Arnold et al., 1986b), g(p) is an isolated simple eigenvalue of Lε(p); is its non-negative eigenfunction and satisfies . For its adjoint operator , is the unique eigenfunction corresponding to g(p) with the property of ; i.e.
Generally, by solving Eq. (12), the moment Lyapunov exponent can be obtained. However, it is impossible so far since the second-order operators are so complicated and is a quaternion function. Therefore, the perturbation method is applied, the asymptotic expressions of g(p) and about ε are given in advance; i.e.
Substituting Eq. (13) into Eq. (12) and equating the terms of the equal powers of ε, the following recursion equations are obtained:
3.1 Solution of zero-order perturbation
According to the first expression of Eq. (14), the zero-order perturbation equation becomes
Because of the property of the moment Lyapunov exponent g(0)=0, we know from Eq. (12) that g0(0)=0. Furthermore, since the left-hand side of Eq. (15) does not contain the variable p, the right side does. Thus, g0(0)=0 yields g0(p)=0; Eq. (15) simplified as
In order to make the problem solvable, it is supposed that θ, φ1, φ2, and ξ are mutually independent. Thus, the measure is assumed that , and substituting it into Eq. (16), we get
Solving the above equation yields , where k1,2 and c1,2 are real constants. Since Φ1(φ1) and Φ2(φ2) are periodic function of φ1 and φ2, respectively, is obtained, and Φ1(φ1) and Φ2(φ2) can be chosen as 1. Hence the differential equation for ψ0(ξ) becomes
Solving the above equation, the solution is
Since ψ0(ξ) is bounded and periodic, C1=0. So ψ0(ξ) is a constant; we let ψ0(ξ)=1. Therefore, the final expression of the measure is as follows:
It is just the joint probability density function of the phase processes .
Applying the above same method, the corresponding adjoint differential equation of Eq. (15) is written as
Its solution that represents the joint probability density function of the independent random variables is obtained:
3.2 Solution of first-order perturbation
From Eq. (14), the differential equation of the first-order perturbation is as follows:
Due to g0(p)=0, Eq. (24) is simplified as
According to Eq. (24), we will seek g1(p) and . The solvability condition of the above expression is
where is given in Eq. (24), and denotes the inner product that is defined as
Solving Eq. (25), the first-order term of the moment Lyapunov exponent is acquired:
and it can be seen from Eq. (20) that , so by a simple calculation, the following expression is derived such that
And , Eq. (27) is rewritten as
Integrating Eq. (28) for ξ from 0 to 2π, we have g1(p)=0.
Thus, Eq. (23) is simplified as
i.e.
For the convenience of writing, we let .
In order to obtain the joint measure , an auxiliary time t′ is introduced in Eq. (30), and it becomes
Through the linear transformation , and , and the partial derivatives to φ1 and φ2 on the left side of Eq. (31) are transformed into
According to Duhamel's principle (Zauderer and Stephen, 1985), the solution for Eq. (32) is given by
where is the solution of the following homogeneous equation:
For solving Eq. (34), we consider the equations
It can be seen that Eq. (35) is Kolmogorov's backward equation for the transition probability function , which is the probability density function of random variable z(t) conditioned on ξ(s), t>s. The transition probability function with Eq. (35) is presented by
By means of Eqs. (34) and (35), the solution for Eq. (34) is given by
where
By substituting Eqs. (37) and (38) into Eq. (33) and via some calculation, is obtained:
Meanwhile, the measure in Eq. (33) is solved by inserting and into Eq. (39) and evaluating the limit .
3.3 Solution of second-order perturbation
According to Eq. (14), the second-order perturbation is rewritten as
The solvability condition of Eq. (40) is
Through the integral for φ1, φ2, and ξ on [0,2π] and the massive calculations, Eq. (41) can be simplified as
Because of the arbitrariness of function , if Eq. (42) holds, the expression in braces must be identically zero, which engenders the eigenvalue problem for the second-order expansion g2(p) of g(p); i.e.
Now, we solve the eigenvalue problem shown in Eq. (43). At the two boundary points and π/2, the eigenfunction F0(θ) satisfies the zero Neumann boundary condition according to the following papers: Namachchivaya et al. (1996) and Namachchivaya (2001). Then, based on Wedig (1988) and Bolotin (1965), F0(θ) is expanded as an orthogonal expression of a Fourier cosine series; i.e.
Substituting the above expansion into Eq. (43), multiplying cos (2nθ) with both sides of the equation, and integrating with respect to θ on , the following equations can be calculated out:
Equation (45) can be transformed into the vector form
where , and its sub-matrix sequence is
Equation (46) shows that g2(p) is the leading eigenvalue of the infinite-order matrix R. Therefore, an infinite eigenvalue sequence of g2(p) is obtained according to Eq. (47). If the sequence converges to a definite value as n→∞, the value is just the second-order approximation of the moment Lyapunov exponent. However, with the increased n, the large-scale calculations emerge and are even beyond computation. Thus, the truncation method for n is applied by the numerical solution.
For example, as n=0, g2(p)=a00. When n=1, the second-order approximation g2(p) is the eigenvalue of the second-order sub-matrix of R. For n=2, the third-order approximation is the eigenvalue of the third-order sub-matrix of R, etc. If the two or more curves of g2(p) are almost coincident with the increase in n, the curve can be regarded as the approximation of g2(p).
It is not possible to solve the analytical expression of the moment Lyapunov exponent from the eigenvalue problem defined in Eq. (46), especially for the high-order matrix R. Therefore, in order to intuitively indicate the validity of this programme, we give the numerical graphs for the sequence of the moment Lyapunov exponent g(p) in Fig. 2. The influences of the different values of noise and system parameters on the moment Lyapunov exponent g(p) and maximal Lyapunov exponent g′(0) are shown in Figs. 3–7.
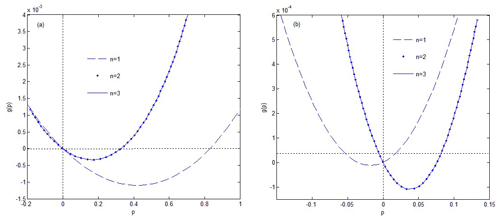
Figure 2Convergence of the moment Lyapunov exponent with the increase in n: (a) , d1=0.1, d2=0.2, A0=1.4, B0=1, , ; (b) , d1=0.1, d2=0.2, A0=0.8, B0=1.2, , , .
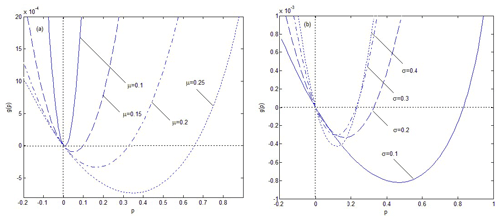
Figure 3Curve variation in the moment Lyapunov exponent with the increase in noise parameters μ and σfor the following case: , d1=0.1, d2=0.2, A0=1.4, B0=1, .
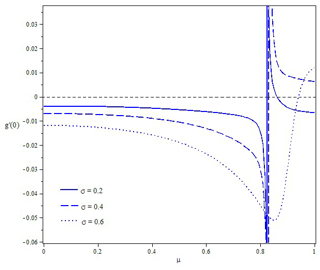
Figure 4Variation in the maximum Lyapunov exponent with noise parameters μ and σ for the following case: , , A0=1.4, B0=1, .
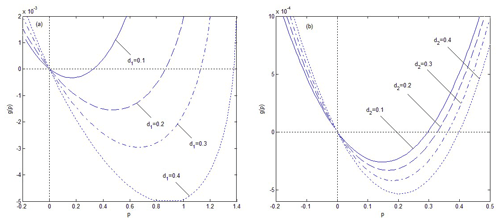
Figure 5Trends of the moment Lyapunov exponent with system parameters d1 and d2 for the following case: , A0=1.4, B0=1, , .
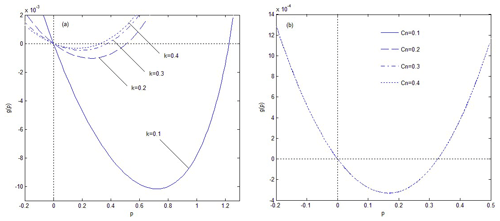
Figure 6Effect of system parameters k and Cn on the moment Lyapunov exponent for the following case: d1=0.1, d2=0.2, A0=1.4, B0=1, , .
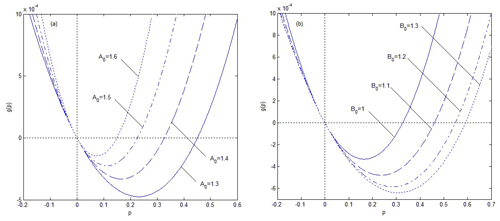
Figure 7Influence of system parameters A0 and B0 on the moment Lyapunov exponent for the following case: , d1=0.1, d2=0.2, , .
In Fig. 2, the curves of the moment Lyapunov exponent g(p) with the increased values of n for two different cases are given. The two pictures display that the deviation of the curves of the moment Lyapunov exponent is very large at n=1 and n=2, where n represents the order of the sub-matrix. However, as n=2 and n=3, the curves of g(p) are nearly coincident. Thus, we conclude that the series of the moment Lyapunov exponent are convergent when the order n of matrix R rises, and it is sufficient for us to truncate the fourth-order approximate of g2(p).
It can be seen from the analytical expressions of the elements in matrix R that the moment Lyapunov exponents are impacted by the noise excitation. In Fig. 3, the curves of the moment Lyapunov exponent with respect to the noise parameters are described. The effects of the drift coefficient μ and diffusion coefficient σ on the moment stability are contrary. The moment stability of the system is weakened with the increase in μ, while it is enhanced with the increased σ. Furthermore, there is a bigger sensitivity near σ=0.1 because the distance of the curves between σ=0.1 and σ=0.2 is larger than among σ=0.2, 0.3 or 0.4. At the same time, the almost sure stability of the system excited by noise is also presented in Fig. 4. When σ=0.2 and 0.4, μ<0.82 and σ=0.6 and μ<0.91, the system is almost surely asymptotically stable. However, jumping between μ=0.82 and μ=0.84, the curves rapidly increase and the system becomes instable, and the trend slightly slows down a little at σ=0.6.
In addition, moment Lyapunov exponents are not only related to noise disturbance but are also affected by system parameters. The effects of the different values of damping coefficients on the moment Lyapunov exponent are shown in Fig. 5. It is obvious that the moment stability of the system is enhanced with the increase in d1 and d2, but the influence of d1 on the system is stronger than that of d2 because the variational intensity of its curves is larger. Figure 6 depicts the moment Lyapunov exponent with the increased k and Cn. The increase in k weakens the system stability, and the larger k is, the smaller the influence becomes from Fig. 6a. In Fig. 6b, all the four curves coincide completely, which indicates that different values of Cn have no effect on the system. Finally, the trends of the curves in Fig. 7a and b are just the opposite of each other, and the moment stability of the system strengthens with the increase in A0.
In this paper, the stochastic stability of a gyro-pendulum system parametrically excited by a bounded noise is investigated through the moment Lyapunov exponent. An eigenvalue problem of the moment Lyapunov exponent is constructed by applying the theory of the stochastic dynamical system. Then, a perturbation method and Fourier cosine series expansion are used to obtain the infinite-order matrix whose leading eigenvalue is just the second-order expansion of the moment Lyapunov exponent. Furthermore, the convergence of the infinite eigenvalue sequence is numerically verified by two typical cases. Finally, the effects of system and noise parameters on the moment Lyapunov exponent are discussed. The impacts of two noise parameters on moment stability are the opposite of each other: the increase in μ makes the stability enhance, and σ has the opposite effect. Among the system parameters, only Cn has no effect on the stability, and moment stability is strengthened with the increased k and A0, while the other parameters weaken it.
The code used during the study can be made available in parts or in its entirety by the corresponding author upon request.
The data used during the study can be made available in parts or in their entirety by the corresponding author upon request.
SL developed the research idea and performed the analysis and simulations. JL discussed the results and prepared the paper. All the authors provided input on the paper for revision before submission.
The contact author has declared that neither of the authors has any competing interests.
Publisher's note: Copernicus Publications remains neutral with regard to jurisdictional claims made in the text, published maps, institutional affiliations, or any other geographical representation in this paper. While Copernicus Publications makes every effort to include appropriate place names, the final responsibility lies with the authors.
The authors acknowledge the financial support of the National Natural Science Foundation of China and the Jiangsu Government Scholarship for Overseas Studies.
This research has been jointly supported by the National Natural Science Foundation of China (grant no. 11602098) and Jiangsu Government Scholarship for Overseas Studies (grant no. JS-2019-231).
This paper was edited by Daniel Condurache and reviewed by two anonymous referees.
Arnold, L.: A formula connecting sample and moment stability of linear stochastic systems, SIAM J. Appl. Math., 44, 793–802, http://www.jstor.org/stable/2101254 (last access: 30 November 2023), 1984.
Arnold, L., Kliemann, W., and Oejeklaus, E.: Lyapunov exponents of linear stochastic systems, in: Lecture Notes in Mathematics, edited by: Arnold, L. and Wihstutz, V., Springer, Berlin, Heidelberg, 1186, 85–125, https://doi.org/10.1007/BFb0076835, 1986a.
Arnold, L., Oeljeklaus, E., and Pardoux, E.: Almost sure and moment stability for linear ito equations, in: Lecture Notes in Mathematics, edited by: Arnold, L. and Wihstutz, V., Springer, Berlin, Heidelberg, 1186, 129–159, https://doi.org/10.1007/BFb0076837, 1986b.
Arnold, L., Doyle, M., and Namachchivaya, N. S.: Small noise expansion of moment Lyapunov exponents for two dimensional systems, Dynam. Stabil. Syst., 12, 187–211, https://doi.org/10.1080/02681119708806244, 1997.
Asokanthan, S. F. and Ariaratnam, S. T.: Almost-sure stability of a gyopendulum subjected to white-noise random support motion, J. Sound Vib., 235, 801812, https://doi.org/10.1006/jsvi.2000.2951, 2000.
Bolotin, V. V.: The Dynamic Stability of Elastic Systems, Am. J. Phys., 33, 752–753, https://doi.org/10.1119/1.1972245, 1965.
Higham, D., Mao, X., and Yuan, C.: Almost sure and moment exponential stability in the numerical simulation of stochastic differential equations, SIAM J. Numer. Anal., 45, 592–609, https://doi.org/10.1137/060658138, 2007.
Hu, D. L., Huang, Y., and Liu, X. B.: Moment Lyapunov exponent and stochastic stability of binary airfoil driven by non-Gaussian colored noise, Nonlinear Dynam., 70, 1847–1859, https://doi.org/10.1007/s11071-012-0577-x, 2012.
Hu, D. L., Huang, Y., and Liu, X. B.: Moment Lyapunov exponent and stochastic stability of binary airfoil under combined harmonic and Gaussian white noise excitation, Nonlinear Dynam., 89, 539–552, https://doi.org/10.1007/s11071-017-3470-9, 2017.
Khasminskii, R. and Moshchuk, N.: Moment Lyapunov exponent and stability index for linear conservative system with small random perturbation, SIAM J. Appl. Math., 58, 245–256, https://doi.org/10.1137/S003613999529589X, 1998.
Kozic, P., Janevski, G., and Pavlovic, R.: Moment Lyapunov Exponent and Stochastic Stability of a Double-beam System Under Compressive Axial loading, Int. J. Solids Struct., 47, 1435–1442, https://doi.org/10.1016/j.ijsolstr.2010.02.005, 2010.
Li, S. H. and Liu, X. B.: The maximal Lyapunov exponent of a co-dimension two-bifurcation system excited by a bounded noise, Acta Mech. Sin., 28, 511–519, https://doi.org/10.1007/s10409-012-0013-y, 2012.
Li, S. H. and Liu, X. B.: Moment Lyapunov exponent for a three dimension system under a real noise excitation, App. math. and mech., 34, 613–626, https://doi.org/10.1007/s10483-013-1695-8, 2013.
Li, S. H. and Wu, J. C.: Stochastic stability and moment Lyapunov exponent for co-dimension two bifurcation system with a bounded noise, J. Vibroeng., 17, 1392–8716, https://www.extrica.com/article/16010/pdf (last access: 18 March 2021), 2015.
Li, S. H. and Xu, Z. R.: Stochastic stability of a planner gyro-pendulum system with synchronous motor driven by Gaussian white noises, Int. J. Struct. Stab. Dy., 19, 1971006, https://doi.org/10.1142/S0219455419710068, 2019.
Li, X. and Liu, X. B.: Moment Lyapunov exponent and stochastic stability for a binary airfoil driven by an ergodic real noise, Nonlinear Dynam., 73, 1601–1614, https://doi.org/10.1007/s11071-013-0888-6, 2013.
Li, X. and Liu, X. B.: Moment Lyapunov Exponent for a Three Dimensional Stochastic System, Chaos Soliton. Fract., 68, 40–47, https://doi.org/10.1016/j.chaos.2014.07.007, 2014.
Liu, X. B. and Liew, K. M.: On the stability properties of a Van der Pol-Duffing oscillator that is driven by a real noise, J. Sound Vib., 285, 27–49, https://doi.org/10.1016/j.jsv.2004.08.008, 2005.
Namachchivaya, N. S.: Stochastic stability of a gyro-pendulum under random vertical support excitation, J. Sound Vib., 119, 363–373, https://doi.org/10.1016/0022-460X(87)90461-5, 1987.
Namachchivaya, N. S.: Moment Lyapunov exponent and stochastic stability of two coupled oscillators driven by real noise, J. Appl. Mech., 68, 903–914, https://doi.org/10.1115/1.1387021, 2001.
Namachchivaya, N. S., Van Roessel, H., and Doyle, M.: Moment Lyapunov exponent for two coupled oscillators driven by real noise, SIAM J. Appl. Math., 56, 1400–1423, https://doi.org/10.1137/S003613999528138X, 1996.
Xie, W. C.: Lyapunov exponents and moment Lyapunov exponents of a two-dimensional near-nilpotent system, J. Appl. Mech.-T. ASME, 68, 453–461, https://doi.org/10.1115/1.1364491, 2001a.
Xie, W. C.: Moment Lyapunov exponents of a two-dimensional system under real noise excitation, J. Sound Vib., 239, 139–155, https://doi.org/10.1006/jsvi.2000.3211, 2001b.
Xie, W. C.: Moment Lyapunov exponents of a two-dimensional system under bounded noise parametric excitation, J. Sound Vib., 263, 593–616, https://doi.org/10.1016/S0022-460X(02)01068-4, 2003.
Zauderer, E. and Stephen, F.: Partial differential equations of applied mathematics, Am. J. Phys., 53, 702–703, https://doi.org/10.1119/1.14293, 1985.
Wedig, W. V.: Analysis and Simulation of Nonlinear Stochastic Systems, in: Nonlinear Dynamics in Engineering Systems, edited by: Schiehlen, W., International Union of Theoretical and Applied Mechanics, Springer, Berlin, Heidelberg, https://doi.org/10.1007/978-3-642-83578-0_42, 1990.
- Abstract
- Introduction
- Gyro-pendulum system excited by a bound noise
- Asymptotic analysis of the moment Lyapunov exponent
- Eigenvalue problem of the moment Lyapunov exponent
- Numerical results of stochastic stability
- Conclusions
- Code availability
- Data availability
- Author contributions
- Competing interests
- Disclaimer
- Acknowledgements
- Financial support
- Review statement
- References
- Abstract
- Introduction
- Gyro-pendulum system excited by a bound noise
- Asymptotic analysis of the moment Lyapunov exponent
- Eigenvalue problem of the moment Lyapunov exponent
- Numerical results of stochastic stability
- Conclusions
- Code availability
- Data availability
- Author contributions
- Competing interests
- Disclaimer
- Acknowledgements
- Financial support
- Review statement
- References